
Drug discovery is the process of finding and developing new medicines that treat or prevent disease. It typically starts with a scientific idea and ends when a new drug reaches the market. However, this journey is far from simple—it’s long, complex, and expensive.
On average, developing a new drug takes 12 to 15 years and can cost more than $1 billion. Researchers begin by identifying a biological target, such as a gene or protein linked to a disease. Next, they search for compounds that can interact with this target in a meaningful way.
Once a few promising compounds are found, scientists optimize them to improve safety, effectiveness, and drug-like properties. Only the best candidate moves forward into clinical trials. From there, the testing continues through multiple phases before the drug is approved for public use. This article will explore each stage of the drug discovery process and how AI is transforming the path to new medicines.
Speed up discovery with PatSnap Eureka AI Agent—your smart partner from concept to market.
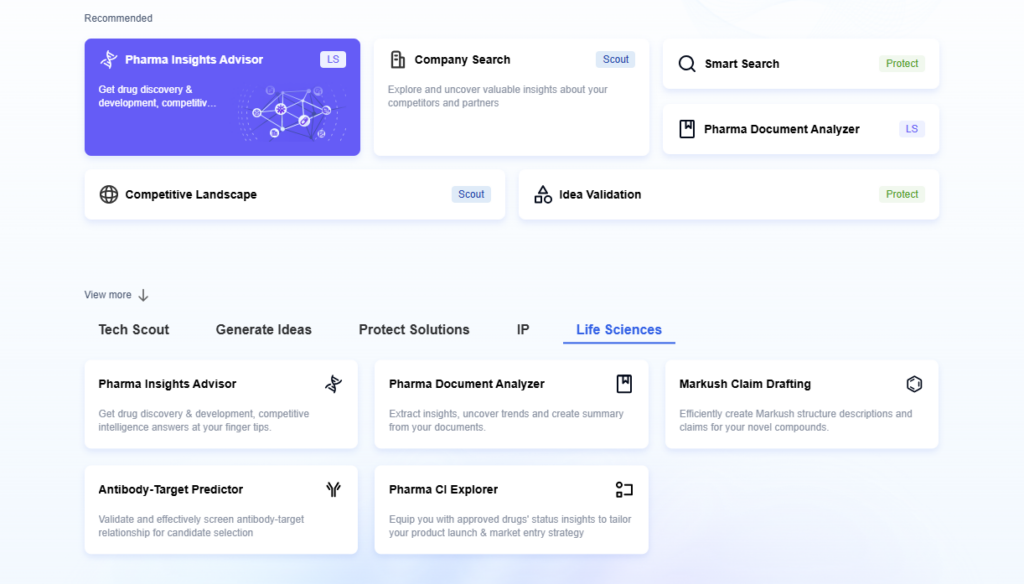
Key Steps in the Drug Discovery Process
The drug discovery process involves several critical stages, each designed to narrow down potential therapies and develop safe, effective medicines.
1. Target Identification and Validation
The drug discovery process begins with target identification, where researchers pinpoint a biological molecule that plays a key role in a disease. These targets are often proteins, receptors, enzymes, or genes. A good target must be druggable, meaning a compound can bind to it and produce a measurable effect in the body.
Once identified, target validation confirms that modulating the target will impact the disease. Scientists use tools like genetic analysis, CRISPR screening, omics data, and bioinformatics platforms to confirm relevance. Functional experiments are also conducted to ensure the target contributes directly to disease progression.
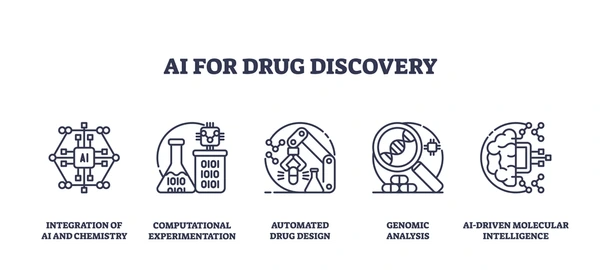
2. Hit Discovery (Compound Screening)
After validating the target, researchers move to hit discovery, where they screen large libraries of chemical or natural compounds to identify those that interact with the target. Hits are molecules that bind to the target and trigger a biological response.
Two main approaches dominate this phase:
- High-throughput screening (HTS): Automated lab systems test thousands of compounds in vitro using biochemical or cell-based assays.
- Virtual screening: Computational models, including AI-driven algorithms, simulate compound-target interactions to predict hits.
A landmark example occurred in 2020 when MIT researchers used a deep-learning AI model to screen over 100 million compounds. The result was halicin, a novel antibiotic that works against multi-drug-resistant bacteria through a previously unknown mechanism.
This phase narrows down thousands of candidates into a handful of promising hits for further development.
3. Lead Optimization
Hit molecules often require modification before they become viable drug candidates. This leads to the lead optimization phase, where chemists refine molecular structures to enhance several key properties:
- Potency: Increase the compound’s ability to interact effectively with the target.
- Selectivity: Minimize off-target effects and unwanted interactions.
- Pharmacokinetics: Improve absorption, distribution, metabolism, and excretion (ADME).
Through iterative testing and structure-activity relationship (SAR) studies, chemists make small chemical changes to fine-tune the compound. AI and machine learning models can now predict how these changes affect performance—reducing the need for countless lab iterations.
Structure-based drug design (SBDD) also plays a crucial role here. By analyzing 3D protein structures, researchers design molecules that fit precisely into the target’s active site.
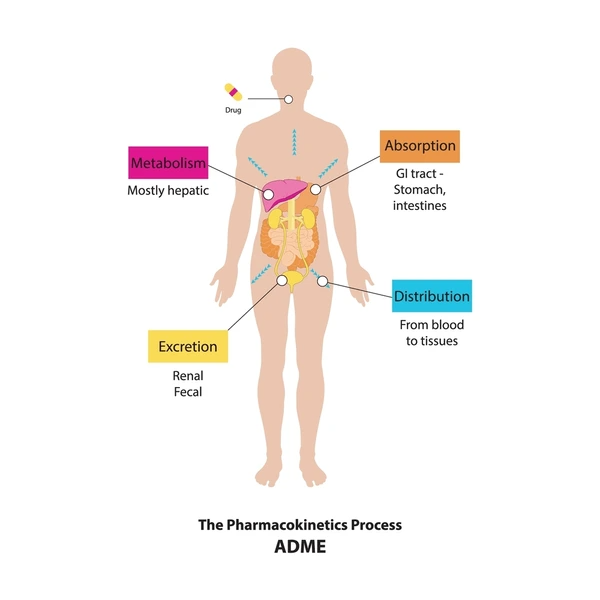
4. Preclinical Testing
Once optimized, a lead candidate undergoes preclinical testing in the lab and in animal models. These studies assess safety, bioavailability, toxicity, and potential side effects. The goal is to ensure the compound has an acceptable safety profile before it enters human trials.
Key preclinical activities include:
- Toxicology studies to identify harmful effects.
- Pharmacokinetic studies to understand how the body processes the drug.
- Efficacy studies in disease models to validate the drug’s intended effect.
AI platforms like Eureka can assist in this stage by predicting toxicity profiles or highlighting adverse interactions based on past preclinical data. These insights help researchers avoid high-risk candidates earlier in the pipeline.
5. Clinical Development
If preclinical studies show promising results, the candidate advances to clinical development, which is divided into three phases:
- Phase I: Small trials on healthy volunteers to evaluate safety and dosage.
- Phase II: Trials on patients to assess efficacy and side effects.
- Phase III: Large-scale trials to confirm effectiveness, monitor adverse reactions, and compare against standard treatments.
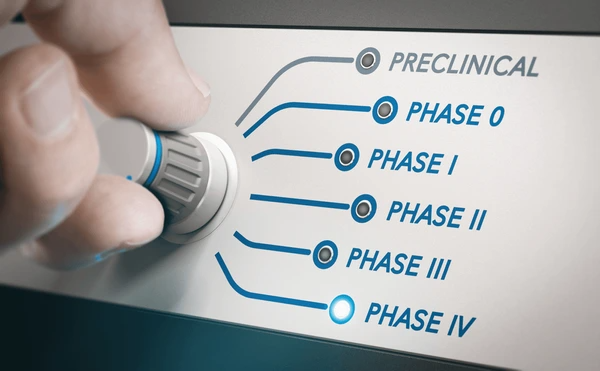
Clinical development is the most expensive and time-consuming stage. Despite careful preparation, around 90% of drug candidates fail during clinical trials, mostly due to efficacy or safety issues.
AI tools and predictive analytics can now support trial design, patient recruitment, and adaptive protocols—helping reduce cost and accelerate decision-making.
Challenges in Drug Discovery
Drug discovery is difficult, and many hurdles can block progress. Key challenges include:
- Time and Cost: Developing a new drug is very expensive and lengthy. It often exceeds a decade and costs over a billion dollars.
- High Failure Rate: Most candidates do not make it to market. About 90% of drugs entering clinical trials fail due to lack of efficacy or safety issues.
- Complex Biology: Diseases often involve complex, poorly understood biology. A target that looks promising in early research may not perform as expected in humans. Predicting how a molecule interacts in the body remains challenging.
- Data Limitations: Modern methods like AI require vast, high-quality data. In drug research, such data are limited. As one review notes, there are billions of images to train AI in vision, but only a few thousand approved drugs to learn from. This data gap can limit the reliability of AI predictions.
- Regulatory Hurdles: Even after successful trials, drugs face strict regulatory review to ensure safety and effectiveness. Meeting these requirements adds time and cost to the process.
Overcoming these challenges requires innovation, teamwork, and often a bit of luck.
Emerging Technologies and Innovations
New technologies are transforming drug discovery. These innovations make research faster and more efficient:
- Artificial Intelligence (AI) & Machine Learning: AI can analyze vast biomedical data and predict molecular behavior. For example, AI-based tools helped discover the antibiotic halicin by screening millions of compounds. AI can also design new molecules and suggest biological targets. However, experts caution that AI is not a silver bullet: it needs high-quality data and careful validation.
- Computational Drug Design: Powerful computers enable virtual screening of huge chemical libraries, finding hits in silico. AI systems like AlphaFold predict protein structures, aiding target selection and drug design. These computational tools complement lab experiments and drastically reduce the search space.
- Genomics and Precision Medicine: Advances in genomics allow identification of disease genes and pathways. Drugs can be tailored to a patient’s genetic profile. For instance, many cancer therapies now target specific genetic mutations.
- Drug Repurposing: Researchers search for new uses of existing drugs. Because these drugs already have known safety profiles, repurposing can be faster and cheaper. For example, thalidomide (originally a sedative) was repurposed for leprosy and cancer. Repurposing projects can sometimes bypass early development stages.
- Advanced Screening Models: Scientists use models like organoids (miniature human organs in dishes) and organ-on-a-chip systems to test drugs. These models can mimic human biology more closely than traditional cell cultures, improving the prediction of a drug’s effects.
- Biologics and New Modalities: The drug pipeline now includes biologics (like monoclonal antibodies), peptides, gene therapies, and mRNA-based treatments. These new modalities often target biological pathways differently and require unique discovery methods.
Many of these technologies work together. For example, AI algorithms analyze data from genomic studies and lab screens. Drug repurposing projects often use computational matching of drugs to diseases. This integration of tools and data is reshaping drug discovery, making it more data-driven and collaborative.
The image above shows bacterial cultures from an AI-driven antibiotic discovery project. A new compound (halicin) kills resistant bacteria in the lower-left dish, while a standard antibiotic does not. This example highlights how AI and virtual screening can reveal novel drug candidates that traditional methods might miss.
Conclusion
Drug discovery is a complex journey from concept to cure. It involves many stages and rigorous testing. Despite challenges like cost and failure rates, new tools are offering hope. Innovations in AI, computation, and biology are accelerating the search for new medicines. As one review notes, AI “has the potential to revolutionize the drug discovery process”. By combining smart technology with scientific expertise and collaboration, researchers aim to bring safer, more effective treatments to patients faster than ever before.
To get detailed scientific explanations of AI-Driven Drug Discovery , try Patsnap Eureka.
