
Drug Discovery and Development Process
Drug discovery and development is a long, complex, and costly journey. Transforming a scientific idea into an approved therapy often takes 10 to 15 years and billions of dollars. Yet despite the challenges, new drugs and vaccines continue to save lives and improve global healthcare. The high stakes make it clear—innovation in this field is not just important, it’s essential.
The process begins in the early discovery phase. Scientists identify a biological target, usually a gene or protein linked to a disease. From there, they may screen thousands of compounds or explore drug repurposing strategies. However, only a small number of these candidates show enough promise to move forward. Out of every 10,000 compounds tested, just 10 to 20 may proceed to the development stage. Of those, only about half enter preclinical testing.
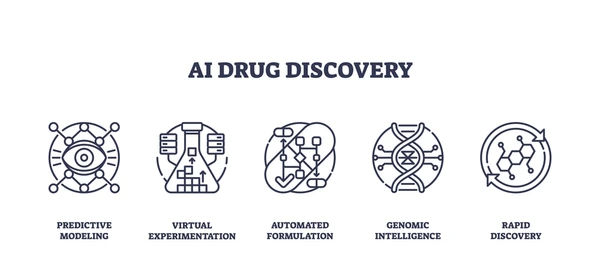
These odds reflect just how high-risk drug development is. Less than 10% of experimental therapies that enter human trials ever gain regulatory approval. This reality adds pressure for researchers to work smarter, faster, and more efficiently.
Several factors contribute to the difficulty. Drug development spans multiple phases—target identification, lead optimization, preclinical studies, clinical trials, and regulatory review. The timeline alone often stretches beyond a decade. At the same time, costs continue to rise, largely because so many candidates fail before reaching the market. Estimates suggest it can take up to $2.6 billion to develop a single approved drug. For instance, only around 7.9% of drugs that start Phase I trials are ever approved.
Given these timelines and risks, the pharmaceutical industry is actively seeking better solutions. Increasingly, researchers are turning to big data and artificial intelligence to reduce uncertainty and accelerate progress. These tools are reshaping the way scientists discover and develop treatments—bringing speed, clarity, and scalability to a process once defined by trial and error.
Accelerate breakthroughs—let PatSnap Eureka AI Agent Guide your Discovery
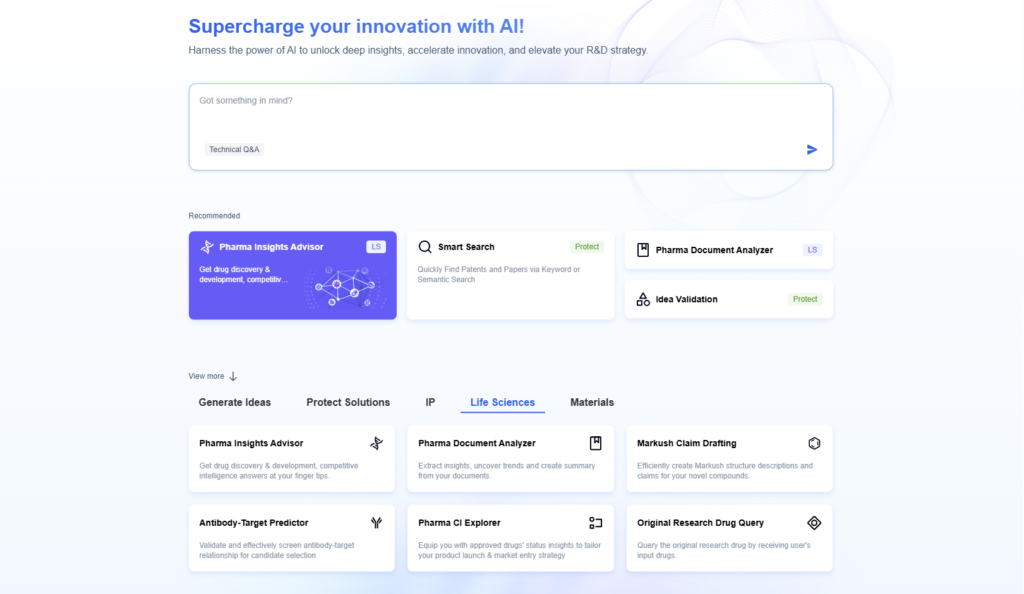
Target Identification and Validation
The first step in drug discovery is identifying a biological target linked to a specific disease. These targets can be proteins, receptors, enzymes, or genes that play a key role in disease progression. Scientists often use genetic research, disease biology, or screening techniques to uncover these targets.
For example, genome-wide association studies (GWAS) can reveal genes connected to a condition. CRISPR screens also help identify essential biological pathways. These methods give researchers a starting point to design therapies with precision.
Traditionally, target discovery involved a combination of lab research and clinical data. Scientists tested thousands of compounds in disease models to identify strong candidates. They also studied rare patients with unique genetic traits to uncover promising targets. As the FDA notes, target discovery requires many tests and deep insights into disease mechanisms.
Out of thousands of leads, only a few move forward. Most fail to show enough relevance or potential. This makes early discovery one of the most resource-intensive stages in drug development.
Now, AI and big data are reshaping this step. Tools powered by GPT and large language models can scan massive biomedical datasets in seconds. These tools read scientific papers, patents, and genetic databases to spot new therapeutic targets.
For instance, GPT-based AI can quickly analyze research to suggest novel disease pathways or biomarkers. It also uncovers patterns that researchers might overlook. By finding shared biological mechanisms, AI supports drug repurposing and combination strategies as well.
These technologies don’t replace lab work—but they speed up early discovery. They help researchers prioritize better leads and avoid time-wasting dead ends. In this way, AI greatly enhances the speed and precision of the target identification process.
Lead Discovery and Optimization
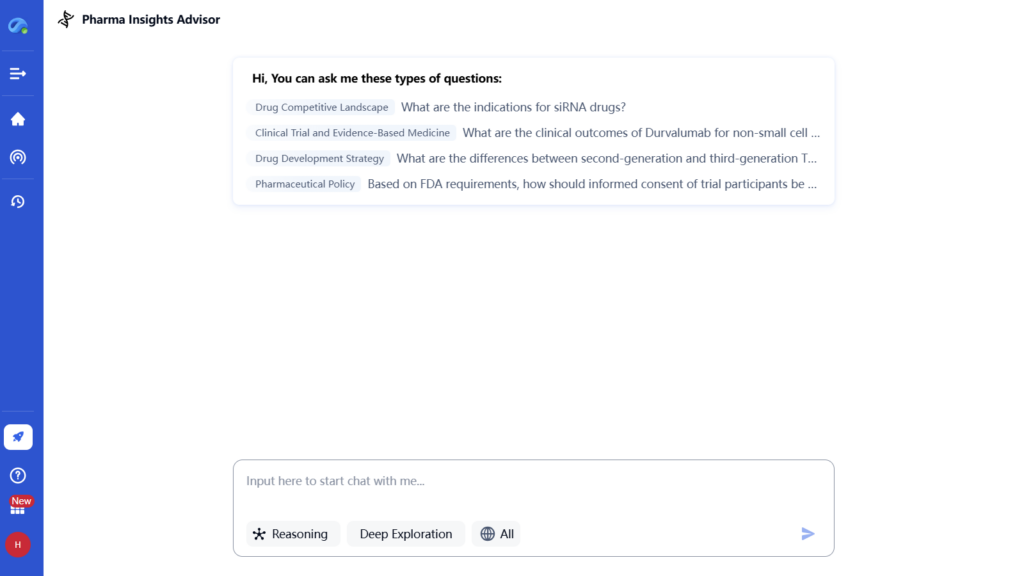
AI Accelerates Lead Discovery in Drug Development
Once scientists validate a target, the next step is lead discovery. Here, researchers search for chemical “hits” that bind to the target and show biological activity. These molecules must interact with the target in a way that could lead to therapeutic benefit.
Traditionally, this process involved building or accessing large chemical libraries. Scientists screened thousands of compounds using cell assays and biochemical tests to identify promising hits. They then refined these molecules through medicinal chemistry, adjusting structures to improve potency, selectivity, and drug-like properties.
This approach takes time. Researchers often test over 10,000 compounds to find just a few usable leads. Each promising hit undergoes multiple rounds of chemical optimization, which can stretch the timeline over several years.
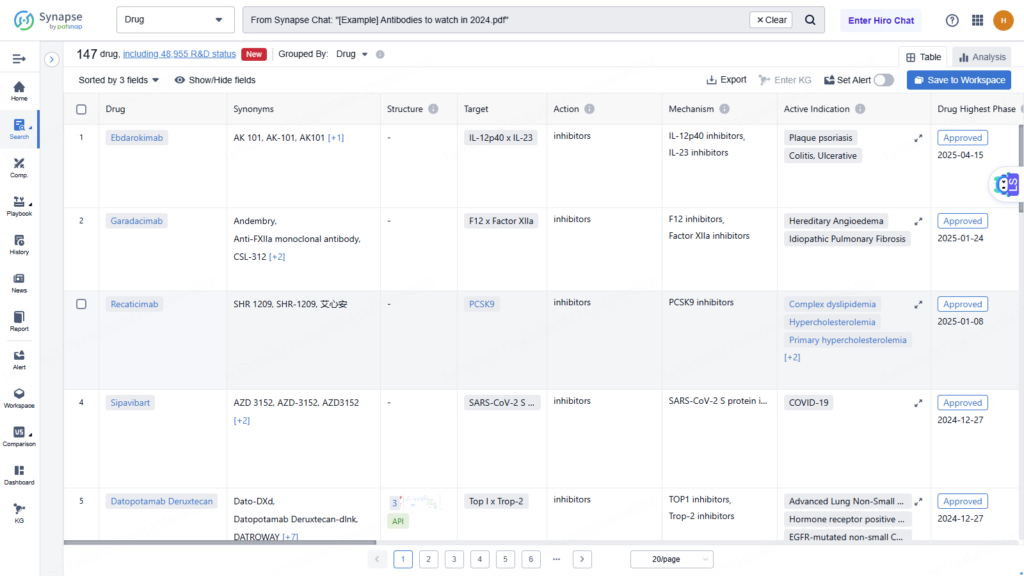
How AI Is Transforming Lead Discovery
Today, AI is speeding up this process dramatically. Instead of physically screening massive libraries, researchers now use virtual chemical libraries and deep-learning models. These models predict which compounds are likely to bind effectively and safely.
In 2020, MIT researchers made headlines using AI to discover a novel antibiotic. Their deep-learning system scanned a virtual chemical space and found halicin, a compound effective against drug-resistant bacteria. This marked a major milestone in AI-driven drug discovery.
Biotech firms like Exscientia and Insilico Medicine are also using AI to design molecules. One AI-designed drug for obsessive-compulsive disorder reached Phase I trials in less than 12 months. That’s far faster than traditional methods, which often take years.
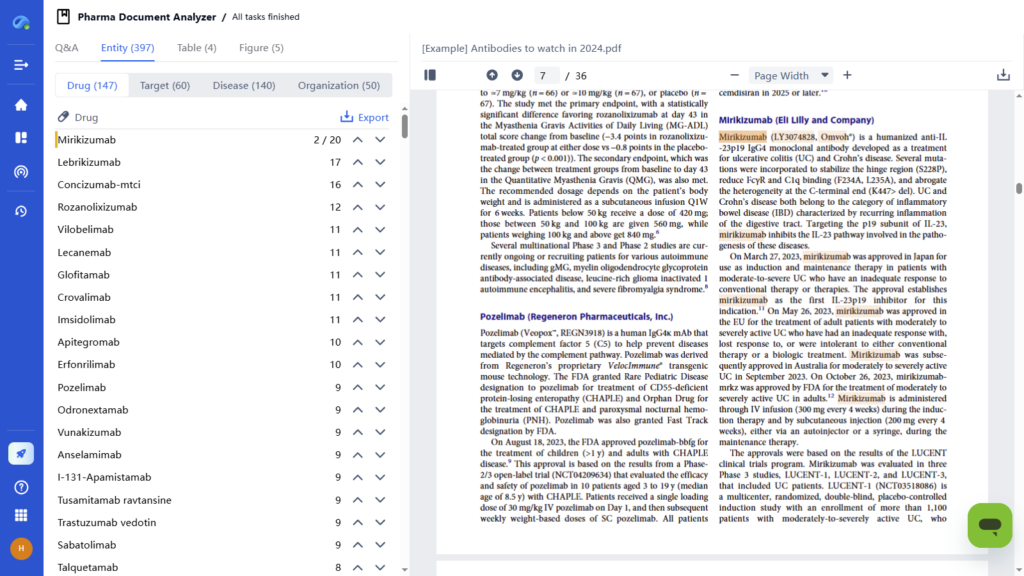
Refining Leads with AI-Powered Optimization
After identifying hits, the next challenge is optimization. Researchers evaluate how the compound behaves in the body. They study absorption, metabolism, toxicity, and delivery methods to ensure safety and effectiveness.
AI helps here, too. Structure-based design uses protein crystal structures to improve how a compound fits its target. Computational models predict which chemical tweaks will enhance performance or reduce side effects—without requiring thousands of lab tests.
This digital approach cuts down the need for guesswork. AI suggests better modifications and quickly filters out weak candidates. As a result, teams spend more time advancing strong leads and less time on failed experiments.
From Trial-and-Error to Intelligent Design
In short, AI shifts lead discovery from physical screening to smart, data-driven design. It scans massive libraries, designs new molecules, and predicts success faster than ever before. Companies like Exscientia now generate novel compounds at record speed, avoiding years of trial-and-error.
This marks a major evolution in drug development. AI doesn’t replace scientists—it empowers them to move faster, explore more possibilities, and deliver better results.et of novel molecules for testing, accelerating what would normally take years of trial-and-error.
Preclinical Testing
After selecting a lead candidate, it enters preclinical development. This stage involves rigorous laboratory testing before any human trials. Scientists conduct in vitro experiments (in cell cultures) and in vivo studies (in animal models) to assess the new compound’s biology, efficacy and toxicity. The goal is to determine if the drug appears safe enough to test in humans.
FDA and other regulators require all preclinical lab work to follow Good Laboratory Practice (GLP) standards. This ensures consistent protocols, data integrity and quality assurance. Preclinical studies typically examine:
- Toxicity: Testing doses in animals to find harmful effects. Doses are increased until adverse reactions appear.
- Pharmacology: Assessing how the drug is absorbed, distributed, metabolized and excreted. Researchers also study how well it treats the disease model.
- Formulation: Developing a stable dosage form (pill, injection, etc.) and establishing the best route of administration.
- Pharmacokinetics/Pharmacodynamics (PK/PD): Measuring blood levels, action on target, and side effects.
These studies can take several years to complete. Only if the preclinical data show an acceptable safety profile will the sponsor apply to start human trials. In the U.S., this means submitting an Investigational New Drug (IND) application to the FDA. The IND includes all preclinical findings, manufacturing details, and proposed clinical protocols. If the FDA approves the IND (often after ~30 days), the drug can move on to Phase I clinical trials.
Emerging methods: Preclinical testing is also being enhanced by new technologies. For example, organ-on-chip systems and 3D tissue cultures aim to predict human toxicity more accurately than animal tests. AI models are used to predict toxicological endpoints from chemical structure, potentially reducing reliance on animal studies. Advances like these can identify failures earlier and refine candidates before costly clinical trials.
Clinical Trials (Phases I–III)
If preclinical results are positive, the drug enters clinical trials—studies in human volunteers—to rigorously assess safety and efficacy. Clinical research is typically divided into three progressive phases:
- Phase I: The first-in-human tests involve a small group of 20–100 healthy volunteers (or patients, for some diseases). These trials focus on safety, tolerability and basic pharmacology. Participants receive the drug at increasing doses while researchers monitor side effects and how the body processes the drug (pharmacokinetics). Phase I establishes the safe dose range for further testing. Trials are tightly controlled and reviewed by ethics boards before starting. AI is beginning to help here by modeling likely safe dose ranges from animal data and human simulations, potentially reducing dose-finding time.
- Phase II: Phase II trials enroll hundreds of patients who have the target disease. These studies test whether the drug has any therapeutic effect on the condition, and continue to monitor safety. Phase II is often randomized and may include a placebo or standard-of-care control group. It helps identify the optimal dose and assesses short-term efficacy signals. According to FDA data, about 70% of drugs successfully complete Phase II and move to Phase III. This high attrition (30% fail) reflects issues like insufficient efficacy or safety concerns that emerge when testing real patients.
- Phase III: These are the pivotal trials with hundreds to thousands of patients. Phase III aims to provide definitive evidence that the drug works (efficacy) and is safe for wider use. Large, multi-center studies compare the new drug against placebo or standard treatment. They gather most of the safety data, identifying rare side effects that smaller trials missed. Phase III trials require extensive coordination (clinicians, statisticians, IRBs, data monitoring). Only about one-third of drugs entering Phase III successfully complete this stage. As PPD notes, “only 12% of drugs make it through this stage” of development. In practice, by the time a drug has passed Phase III, overall approval success rates rise but remain modest.
The clinical trial phases progress from early safety (Phase I) through preliminary efficacy (Phase II) to large-scale confirmation (Phase III). Each phase has distinct goals and enrollment criteria. Key trial characteristics (controlled vs. open-label, blinded vs. open, randomization) are chosen to minimize bias and maximize data quality. The entire clinical phase can last 5–10 years depending on the disease and enrollment pace.
Innovation in trials: New approaches are changing how trials are run. For example, adaptive trial designs use interim data to adjust dosing or patient allocation without halting the study. Decentralized and virtual trials leverage telemedicine, wearable devices and home health monitoring to recruit and follow patients more quickly. AI and analytics help identify ideal trial sites and patient subgroups, improving enrollment and efficiency. Some cutting-edge platforms even use AI to simulate clinical trials: for instance, QuantHealth reports it can predict Phase II outcomes with ~88% accuracy by training on historical trial data. These tools promise to reduce wasted time and cost in clinical development.
Regulatory Approval
After successful Phase III trials, the sponsor compiles all data for regulatory review. In the U.S., this means submitting a New Drug Application (NDA) or Biologics License Application (BLA) to the FDA. A BLA is required for biological products (e.g. monoclonal antibodies, enzymes, vaccines). The application package is comprehensive: it includes all preclinical and clinical study reports, statistical analyses, proposed labeling, manufacturing details, patent and exclusivity information, and more. The goal is to demonstrate that the drug is safe and effective for its intended use.
Regulators then evaluate the submission and may approve the drug, ask for more information, or in rare cases refuse. The FDA review process can follow different timelines: a standard review takes about 10–12 months, but there are expedited pathways (Priority Review, Fast Track, Breakthrough Therapy, Accelerated Approval) for drugs that meet urgent unmet needs. For example, during the COVID-19 pandemic, the FDA issued Emergency Use Authorizations (EUAs) to allow vaccine and treatment use before full approval.
If approved, the drug is cleared for marketing. Companies can then manufacture at commercial scale (often hundreds to thousands of kilograms) under Good Manufacturing Practice (GMP) quality systems. Labels and packaging are finalized, and distribution channels are set up. The regulatory authorities (FDA, EMA in Europe, PMDA in Japan, etc.) continue to monitor the process to ensure quality and compliance.
Post-Market Surveillance (Phase IV)
Approval is not the end of development. Post-market surveillance (sometimes called Phase IV) continues to assess a drug’s performance in the real world. As millions of patients use the therapy, more data on safety and effectiveness emerge. The FDA and other agencies require ongoing monitoring of adverse events via databases (e.g. FDA’s FAERS database). Manufacturers often conduct post-approval studies to explore long-term effects, quality-of-life outcomes, or use in specific populations.
Data collected post-approval can reveal rare or delayed side effects not seen in trials. If safety signals arise, regulators may update labeling (adding warnings), restrict usage, or even withdraw a drug. The true benefit-risk profile of a drug often becomes clearer only after years on the market. Companies also pursue additional approvals (e.g. for new indications or patient groups) by submitting supplemental applications with new data.
Innovations in this phase include using AI and machine learning to sift electronic health records, social media, and global health databases for safety patterns. For example, AI algorithms can flag potential adverse drug reactions by analyzing millions of clinical notes or lab reports in near-real-time. This proactive pharmacovigilance shortens the feedback loop between drug use and detection of problems. In effect, post-market surveillance becomes a data-driven process, as crucial to the drug’s lifecycle as the pre-approval studies.
AI and Emerging Technologies in Drug Development
Modern drug development increasingly relies on pharma innovation and technology. Artificial intelligence (AI), machine learning (ML), and big data analytics are transforming many stages of the drug discovery process. These tools can analyze massive datasets (genomic data, chemical libraries, published literature, patents, clinical trial results, etc.) far beyond human capacity.
In summary, AI in pharmaceutical research is enabling a shift from intuition-driven R&D to data-driven innovation. It helps scientists discover leads faster, optimize candidates more intelligently, and navigate regulatory and competitive landscapes with greater confidence. In practice, AI is now a core part of many drug pipelines. Companies like Insilico, Exscientia, BenevolentAI, and Cyclica are forging new models (sometimes offering AI as a service) that resemble software platform businesses for drug discovery. This “pharma innovation” revolution is still young, but growing rapidly: hundreds of drug programs now use AI, and dozens have entered clinical trials.
Trends, Challenges, and Future Outlook
While technology improves efficiency, cost, time, and failure rates remain major challenges in drug development. According to industry data, developing a new drug can cost over $1–3 billion. Much of that expense arises from late-stage failures. As noted, only roughly 8% of drugs in Phase I make it through the pipeline. The average total time from discovery to market is still on the order of a decade, despite efforts to accelerate it. For context, U.S. patents last 20 years, so much of a drug’s patent life is spent in development.
Current trends
- Accelerated Approvals: Regulatory agencies increasingly offer fast-track and breakthrough designations to get vital therapies to patients sooner. The COVID-19 vaccine programs showed that, under extraordinary circumstances, timelines can be compressed to under a year.
- Personalized Medicine: An explosion of genomics means drugs can be tailored to patient subgroups (e.g. biomarker-driven oncology drugs). While this can improve success rates for targeted therapies, it also makes trials more complex and competitive.
- Digital and Decentralized Trials: The pandemic taught the industry that remote monitoring and virtual consent are feasible. These methods broaden access to trials and can speed enrollment.
- Manufacturing Innovations: Complex biologics and gene therapies challenge manufacturing. Advances like continuous bioprocessing and 3D bioprinting of tissues (as scaffolds) are emerging.
- Globalization: Drug development is increasingly global, with trials and regulatory interactions across the US, Europe, China, India and elsewhere. This requires harmonizing standards but also offers broader patient pools.
Challenges ahead: Despite optimism, many hurdles remain. High failure rates in Phase II (around 30–40%) persist, meaning better preclinical models and biomarkers are needed. Costs continue rising; thus, demonstrating value (especially in the face of drug pricing pressure) is crucial. Ethical issues around AI (data privacy, bias) and regulatory acceptance of AI-driven decisions are emerging concerns. Moreover, the “last mile” of approval – scaling manufacturing and market access – remains a non-trivial task.
Nonetheless, the outlook is promising. As one report notes, AI “is transforming the landscape of drug discovery” by enabling more efficient target identification and decision-making. PatSnap’s Eureka and similar platforms represent a new layer of knowledge infrastructure for R&D. In the near future, we may see hybrid human-AI teams where researchers use intelligent assistants at every step.
Aspect | Traditional Approach | AI-Driven Approach |
---|---|---|
Target Identification | Experimental screening, manual literature review | AI/ML models and NLP to scan literature and data, uncover new targets |
Lead Discovery | High-throughput lab screens of physical compounds | Virtual screening and generative design (e.g. Exscientia, Insilico) to propose novel molecules |
Preclinical Evaluation | In vitro/in vivo studies (animals, GLP studies) | Predictive toxicology models, organ-on-chip, in silico ADMET predictions |
Clinical Trials Design | Protocol set by expert committees | AI-driven adaptive designs; algorithms for site/patient selection |
Data Analysis/IP | Manual analysis of trial results and patents | Automated mining of publications/patents with AI; e.g. Eureka scans sequences in minutes |
Time to Clinic | Years or decades | Reduced by ~30–50% in early stages (some AI-designed drugs entered trials in ~1 year) |
Cost of Discovery | ~$1–3+ billion per approved drug | Potentially 20–30% lower (PatSnap reports ~25% cost reduction) |
Success Rate | ~1 in 10 candidates reaches market | Early signals of higher Phase I success (~80–90% vs. ~40–65% historically) |
Conclusion
The journey from laboratory bench to bedside involves many stages: identifying a biological target, discovering and optimizing compounds, conducting rigorous preclinical and clinical tests, and navigating regulatory approval and post-market monitoring. Each stage has its challenges, from high attrition to complex manufacturing.
Thanks to digital innovation, the landscape of drug discovery is evolving. Artificial intelligence and data-driven tools—exemplified by platforms like PatSnap’s Eureka—are streamlining research. They enable faster hypothesis generation, smarter candidate selection, and more efficient regulatory strategy. Current trends such as precision medicine, accelerated approval pathways, and global collaboration are also reshaping development.
However, the core mission remains the same: to deliver safe and effective new therapies to patients. By integrating cutting-edge technology with rigorous science, the pharmaceutical industry aims to shorten timelines, cut costs, and improve success rates. As one analyst put it, embracing AI and innovation can “usher in an era of more efficient and targeted drug discovery”, bringing hope to patients worldwide.
To get detailed scientific explanations of Drug Discovery, try Patsnap Eureka.
