
Data analysis is a crucial component of research that helps transform raw data into meaningful insights, guiding decisions and advancing knowledge. It involves the systematic application of statistical and logical techniques to describe, summarize, and evaluate data, ensuring that findings are accurate and actionable.
The process of data analysis varies depending on the type of research and the data at hand. Whether you’re dealing with qualitative insights or quantitative measures, having a solid understanding of data analysis methods is essential for producing valid, reliable results. In this guide, we will explore the different methods and types of data analysis, their importance in research, and how integrating AI tools can enhance the data analysis process.
What is Data Analysis in Research?
Curious about how AI is transforming data analysis? Eureka Technical Q&A connects you with AI and data science experts who can explain how machine learning algorithms, automation, and predictive models are optimizing data workflows—giving you the insights needed to leverage AI in your own analysis processes.
At its core, data analysis in research involves inspecting, cleaning, transforming, and modeling data to extract useful information, inform conclusions, and support decision-making. It is a foundational process in research that ensures findings are not just random occurrences but are based on rigorous analysis of data. Through data analysis, researchers are able to draw meaningful conclusions from complex datasets, leading to valid, generalizable insights.
Data analysis can involve both qualitative and quantitative approaches, each contributing to different aspects of research. The proper choice of method depends on the research question, data type, and the specific goals of the study.
Types of Data Analysis Methods
Different types of research and data call for distinct methods of analysis. The two primary categories of data analysis are qualitative and quantitative analysis, each with its own set of techniques.
1. Qualitative Data Analysis
Qualitative data analysis focuses on non-numeric data, aiming to understand concepts, experiences, or behaviors through patterns and themes. The methods used in qualitative analysis emphasize the richness and depth of data, often in the form of text, images, or audio recordings.
Common methods include:
- Content Analysis: Examining texts, media, or communications to identify themes or patterns.
- Thematic Analysis: Identifying and analyzing recurring themes within qualitative data.
- Narrative Analysis: Studying personal stories or accounts to understand how individuals interpret events.
These methods are often used in fields such as psychology, sociology, and anthropology, where understanding the meaning behind human behavior is the focus of the research.
2. Quantitative Data Analysis
Quantitative analysis deals with numerical data and applies statistical methods to examine relationships, test hypotheses, and measure variables. This type of analysis allows researchers to make inferences and generalize findings from sample data to larger populations.
Common techniques include:
- Descriptive Statistics: Summarizing data to describe its main characteristics, such as mean, median, and standard deviation.
- Inferential Statistics: Using sample data to make inferences or test hypotheses about a population, including regression analysis and hypothesis testing.
- Predictive Analytics: Employing statistical models and machine learning techniques to forecast future trends based on historical data.
These methods are essential for large-scale studies, providing concrete, quantifiable results that can guide future research or inform real-world applications.
The Importance of Data Analysis in Research
Data analysis is critical for several reasons in the research process:
- Informed Decision-Making: It provides the foundation for evidence-based decisions, whether in science, business, or policy-making.
- Identifying Trends and Patterns: Through analysis, researchers can uncover trends, correlations, and anomalies that help understand complex phenomena.
- Enhancing Research Quality: Proper data analysis ensures that research findings are valid, reliable, and scientifically robust, contributing to the credibility of the study.
- Supporting Hypothesis Testing: Data analysis allows researchers to test hypotheses and validate theoretical frameworks, driving scientific progress.
In short, data analysis helps to answer research questions with confidence and clarity, making it a fundamental step in any research study.
How AI Enhances the Data Analysis Process
With the increasing complexity of data in modern research, AI-powered tools like Eureka AI Agent are transforming data analysis by making it faster, more accurate, and scalable. Eureka AI Agent offers a wide range of capabilities that enhance various aspects of the data analysis process.
1. Data Integration and Collection
Eureka AI Agent can seamlessly integrate data from multiple sources, bringing together information from diverse databases, platforms, and formats. This comprehensive data collection ensures that researchers have all relevant information at their disposal, saving time and effort in the initial stages of data analysis.
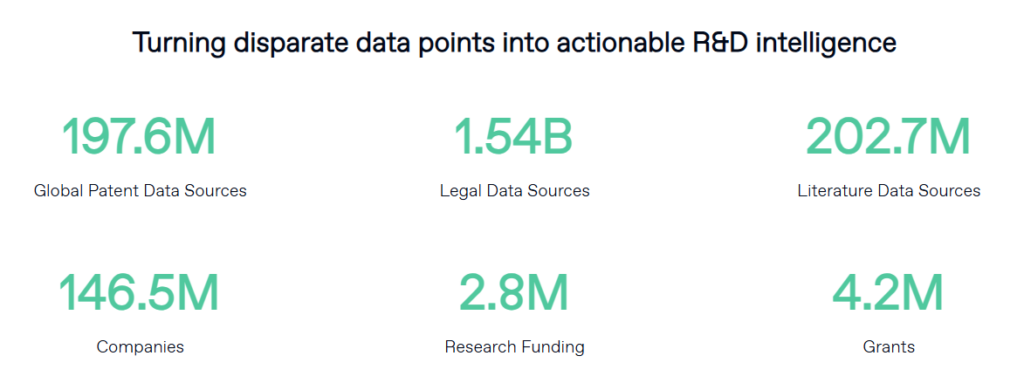
2. Anomaly Detection
One of the key challenges in data analysis is identifying errors or outliers in large datasets. Eureka AI uses advanced algorithms to automatically detect anomalies, helping researchers spot data issues early on and ensuring more reliable results.
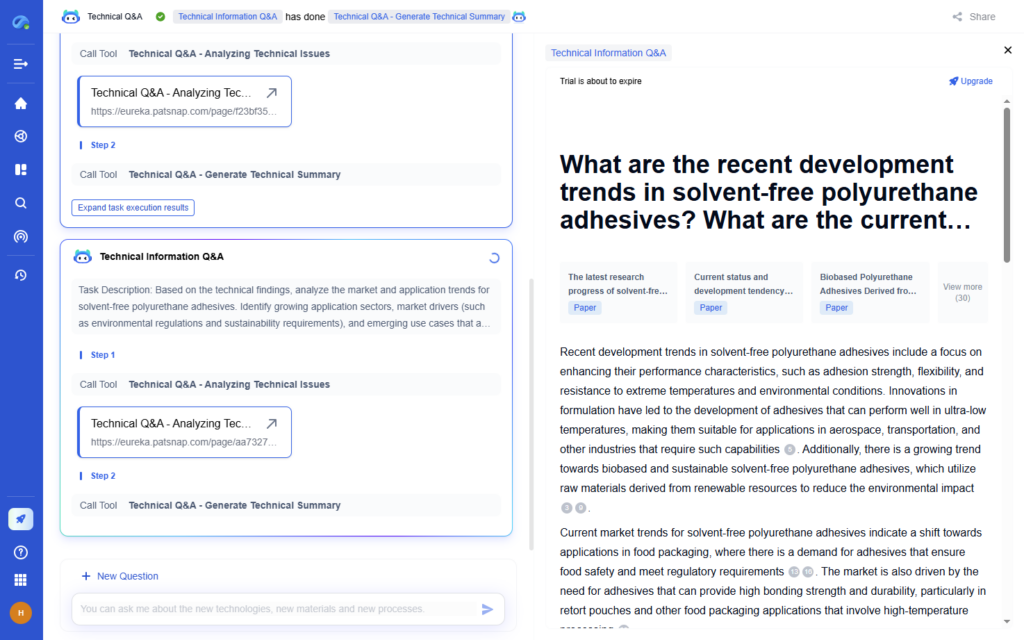
3. Predictive Analytics
Eureka AI Agent leverages machine learning models to forecast trends based on historical data, helping researchers predict outcomes and identify patterns that may not be obvious from raw data alone. This predictive capability is particularly useful in fields such as marketing, healthcare, and finance.
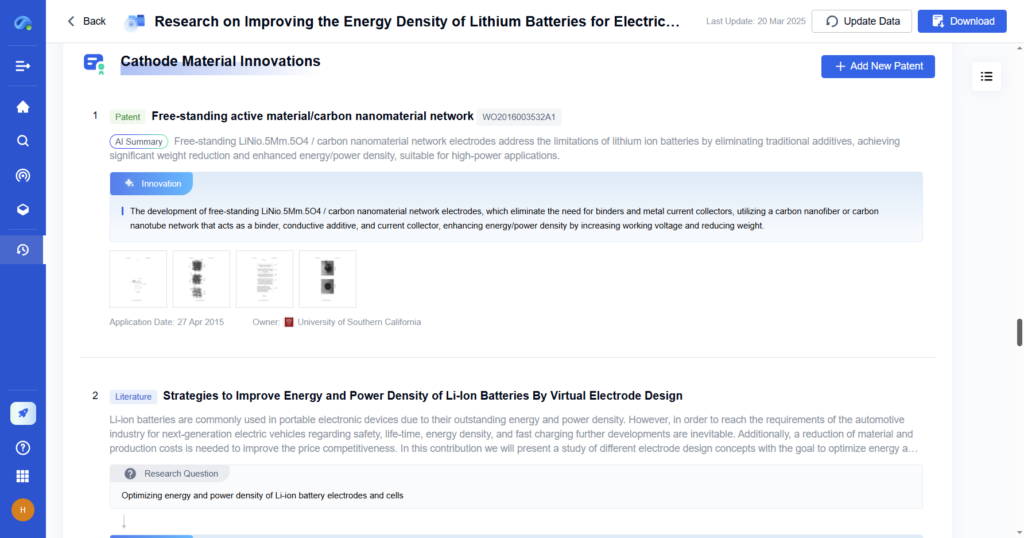
4. Text and Data Mining
For research that involves large volumes of unstructured data (such as text documents or multimedia), Eureka AI’s natural language processing (NLP) and data mining capabilities allow researchers to extract relevant information and identify key insights from qualitative data.
5. Real-Time Data Analysis
Eureka AI provides real-time analytics, allowing researchers to analyze data as it is collected, making the research process more agile and allowing for quicker adjustments and decisions.
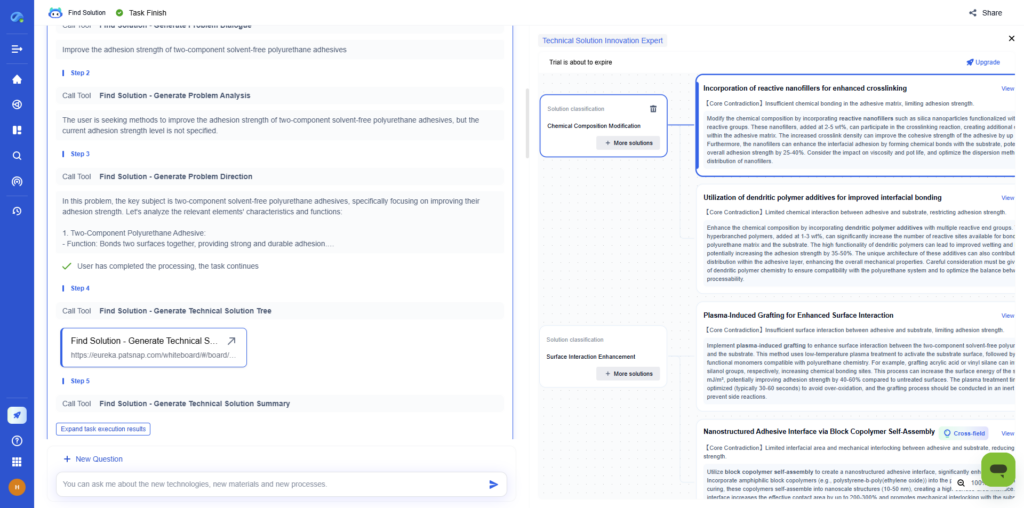
6. Report Generation and Visualization
After completing the analysis, Eureka AI automatically generates structured reports and visualizations, summarizing key findings and trends. These visual tools, such as correlation matrices or trend charts, help researchers present data in a more digestible format for stakeholders, ensuring better communication of results.
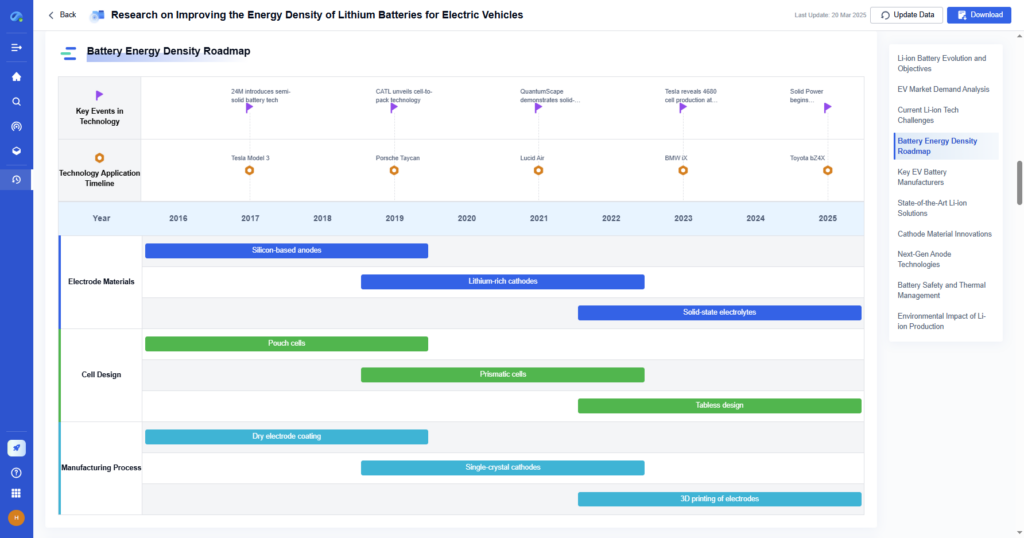
Conclusion
Data analysis is the backbone of research, providing the necessary tools to transform raw data into valuable insights. Whether using qualitative or quantitative methods, the importance of data analysis cannot be overstated. It enables researchers to uncover hidden patterns, support hypothesis testing, and make informed decisions.
Incorporating AI tools like Eureka AI Agent into data analysis helps researchers speed up their work. It also uncovers deeper insights and generates reports automatically. This integration ensures both accuracy and reliability. As data becomes more complex and voluminous, using AI is no longer just a benefit. It’s now essential for advancing high-quality research across various disciplines.
To get detailed scientific explanations of Data Analysis in Research, try Patsnap Eureka.
