Hyperspectral Image Classification Method Based on 3D Lightweight Deep Network
A hyperspectral image and deep network technology, applied in the field of hyperspectral image classification, can solve the problem of shallow deep learning model, and achieve the effect of deep network model, less parameters and high precision
- Summary
- Abstract
- Description
- Claims
- Application Information
AI Technical Summary
Problems solved by technology
Method used
Image
Examples
Embodiment Construction
[0026] Now in conjunction with embodiment, accompanying drawing, the present invention will be further described:
[0027] The technical solution of the present invention is a hyperspectral image classification method based on a three-dimensional lightweight deep network. This method extracts a small number of labeled samples from the hyperspectral image to be processed to train the three-dimensional lightweight deep network proposed in this technical solution, and then uses the trained network model to classify the entire set of images.
[0028] The concrete measures of this technical scheme are as follows:
[0029] Step 1: Data preprocessing. The hyperspectral image data to be processed is subjected to maximum and minimum normalization.
[0030] Step 2: Data partitioning. Count the number of labeled samples of each category in the hyperspectral image to be processed, and then extract 5%-10% of the labeled samples from each category in proportion as training data, and the ...
PUM
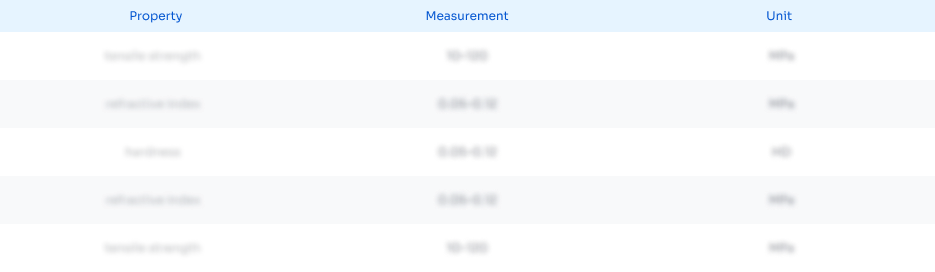
Abstract
Description
Claims
Application Information
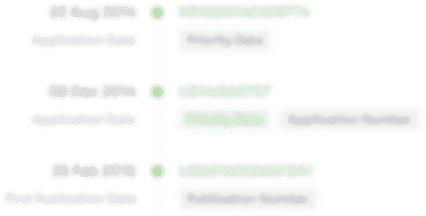
- R&D
- Intellectual Property
- Life Sciences
- Materials
- Tech Scout
- Unparalleled Data Quality
- Higher Quality Content
- 60% Fewer Hallucinations
Browse by: Latest US Patents, China's latest patents, Technical Efficacy Thesaurus, Application Domain, Technology Topic, Popular Technical Reports.
© 2025 PatSnap. All rights reserved.Legal|Privacy policy|Modern Slavery Act Transparency Statement|Sitemap|About US| Contact US: help@patsnap.com