Compressive attention model for semantic segmentation of pixel group
An attention model and semantic segmentation technology, applied in biological neural network models, character and pattern recognition, instruments, etc., to achieve the effect of enhancing pixel-level dense prediction and good semantic segmentation effect
- Summary
- Abstract
- Description
- Claims
- Application Information
AI Technical Summary
Problems solved by technology
Method used
Image
Examples
Embodiment Construction
[0022] The specific implementation of the present invention will be described in detail below in conjunction with the accompanying drawings. As a part of this specification, the principles of the present invention will be described through examples. Other aspects, features and advantages of the present invention will become clear through the detailed description. In the referenced drawings, the same reference numerals are used for the same or similar components in different drawings.
[0023] Such as figure 1 As shown, the compressed attention model for semantically segmented pixel groups of the present invention includes:
[0024] To learn more representative features for the semantic segmentation task through a reweighting mechanism that considers both local and global aspects.
[0025] The SA model first uses the residual network (ResNets) as the basic residual block. The traditional residual representation is as (1):
[0026]
[0027] F( ) represents the residual func...
PUM
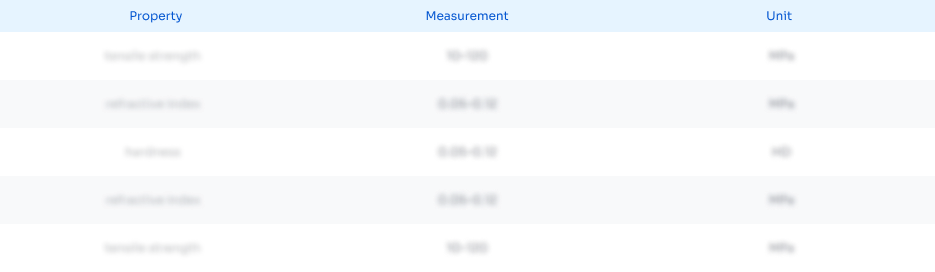
Abstract
Description
Claims
Application Information
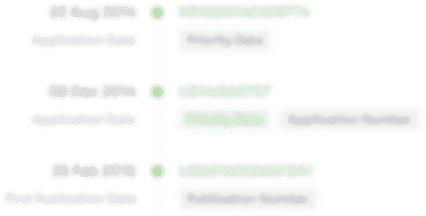
- Generate Ideas
- Intellectual Property
- Life Sciences
- Materials
- Tech Scout
- Unparalleled Data Quality
- Higher Quality Content
- 60% Fewer Hallucinations
Browse by: Latest US Patents, China's latest patents, Technical Efficacy Thesaurus, Application Domain, Technology Topic, Popular Technical Reports.
© 2025 PatSnap. All rights reserved.Legal|Privacy policy|Modern Slavery Act Transparency Statement|Sitemap|About US| Contact US: help@patsnap.com