A deep learning-based thermal error modeling method for machine tools
A technology of deep learning and thermal error, applied in the field of CNC machine tools, can solve the problems of high-dimensional data dimension disaster, restricting thermal error compensation technology, and difficulty in meeting the robustness requirements of thermal error models, achieving good robustness and prediction high precision effect
- Summary
- Abstract
- Description
- Claims
- Application Information
AI Technical Summary
Problems solved by technology
Method used
Image
Examples
Embodiment Construction
[0028] The present invention will be described in further detail below in conjunction with embodiment.
[0029] 1. Modeling principle of thermal error of gantry five-sided machining center based on SAE-GA-BP
[0030] The principle of the thermal error model is as follows figure 1 As shown, firstly, the temperature data are normalized, and the measuring points that have a large correlation with the thermal errors in the three directions of the main axis are calculated by the partial correlation coefficient method. Then, the temperature data of the key measuring points are input into the stacked auto-encoder (Stacked Auto-Encoder, SAE) neural network as an independent variable, and the corresponding features of the temperature data are extracted. Finally, the temperature feature is used as an independent variable, and the corresponding thermal error data is input into the GA-BP neural network as a dependent variable for training and thermal error prediction.
[0031] 2. Mining...
PUM
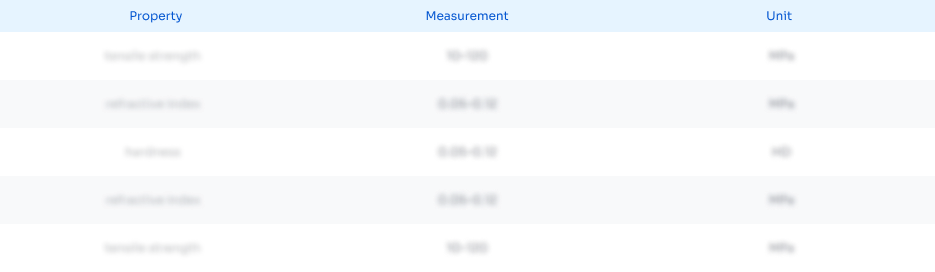
Abstract
Description
Claims
Application Information
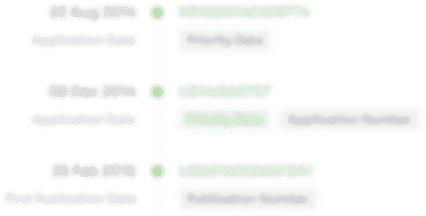
- R&D Engineer
- R&D Manager
- IP Professional
- Industry Leading Data Capabilities
- Powerful AI technology
- Patent DNA Extraction
Browse by: Latest US Patents, China's latest patents, Technical Efficacy Thesaurus, Application Domain, Technology Topic, Popular Technical Reports.
© 2024 PatSnap. All rights reserved.Legal|Privacy policy|Modern Slavery Act Transparency Statement|Sitemap|About US| Contact US: help@patsnap.com