Method and device for training and using relational network embedding model
A relational network and relational technology, applied in the field of relational network and graph embedding, can solve the problems that other features cannot be integrated, the risk control model cannot fully utilize data, and the credit evaluation is low
- Summary
- Abstract
- Description
- Claims
- Application Information
AI Technical Summary
Problems solved by technology
Method used
Image
Examples
Embodiment Construction
[0075] The solutions provided in this specification will be described below in conjunction with the accompanying drawings.
[0076] As mentioned earlier, in credit evaluation, it is often difficult to conduct a comprehensive evaluation due to incomplete and rich user data. For such "thin data" users, in order to perform better credit evaluation, according to the embodiments of this specification, the user's relationship network is used to increase the richness of their data. In general, it can be considered that the user's network can reflect some of his characteristics (such as income, spending power, education, etc.) to a certain extent, so the data of "friends" can be used as one of the dimensions of user credit scoring to participate in the evaluation model.
[0077] Based on the above considerations, according to one or more embodiments of this specification, a comprehensive relationship network is constructed for user credit evaluation. figure 1 is a schematic diagram o...
PUM
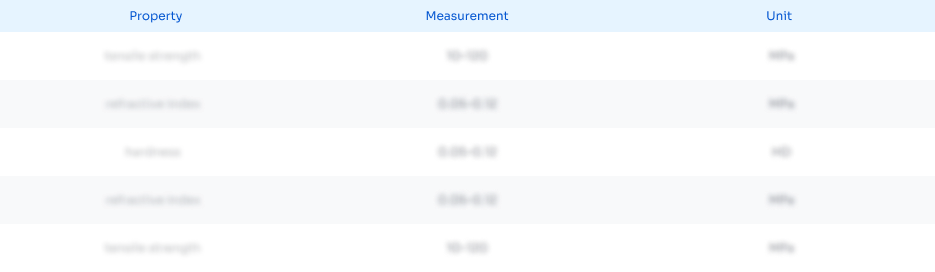
Abstract
Description
Claims
Application Information
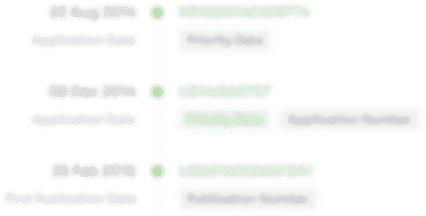
- R&D Engineer
- R&D Manager
- IP Professional
- Industry Leading Data Capabilities
- Powerful AI technology
- Patent DNA Extraction
Browse by: Latest US Patents, China's latest patents, Technical Efficacy Thesaurus, Application Domain, Technology Topic.
© 2024 PatSnap. All rights reserved.Legal|Privacy policy|Modern Slavery Act Transparency Statement|Sitemap