Image classification method and system based on neural network optimization of gradient direction parameters
A gradient direction, neural network technology, applied in the field of neural network image classification, can solve problems such as large amount of calculation and storage, increasing complexity, slow algorithm convergence speed, etc., to achieve good optimization performance, fast decline speed, optimization speed quick effect
- Summary
- Abstract
- Description
- Claims
- Application Information
AI Technical Summary
Problems solved by technology
Method used
Image
Examples
Embodiment 1
[0040] This embodiment provides an image classification method based on a neural network optimized for gradient direction parameters;
[0041] Image classification methods based on neural network optimization of gradient direction parameters, including:
[0042] S101: Acquire an image data set, and preprocess each image in the image data set;
[0043] S102: Build a convolutional neural network CNN, set the hyperparameters and loss functions of the convolutional neural network CNN; use the preprocessed image and the known image classification label as the input value of the convolutional neural network CNN, and start the convolutional neural network CNN. The network CNN is trained; according to the output of the convolutional neural network CNN and the image label, the loss function is calculated, and then the error is back propagated;
[0044] S103: In the process of error back-propagation, the gradient direction parameter optimization method is used to update the parameters ...
Embodiment 2
[0110] This embodiment provides an image classification system based on a neural network optimized for gradient direction parameters;
[0111] An image classification system based on a neural network optimized for gradient direction parameters, including:
[0112] a preprocessing module, which is configured to: acquire an image dataset, and preprocess each image in the image dataset;
[0113] The hyperparameter setting module is configured to: construct a convolutional neural network CNN, set the hyperparameters and loss functions of the convolutional neural network CNN; use the preprocessed image and the known image classification label as the convolutional neural network CNN. Input the value, start training the convolutional neural network CNN; calculate the loss function according to the output of the convolutional neural network CNN and the image label, and then perform the error back propagation;
[0114] The gradient direction parameter optimization module is configured...
Embodiment 3
[0120] This embodiment also provides an electronic device, comprising: one or more processors, one or more memories, and one or more computer programs; wherein the processor is connected to the memory, and the one or more computer programs are Stored in the memory, when the electronic device runs, the processor executes one or more computer programs stored in the memory, so that the electronic device executes the method described in the first embodiment.
[0121] It should be understood that, in this embodiment, the processor may be a central processing unit CPU, and the processor may also be other general-purpose processors, digital signal processors DSP, application-specific integrated circuits ASIC, off-the-shelf programmable gate array FPGA or other programmable logic devices , discrete gate or transistor logic devices, discrete hardware components, etc. A general purpose processor may be a microprocessor or the processor may be any conventional processor or the like.
[...
PUM
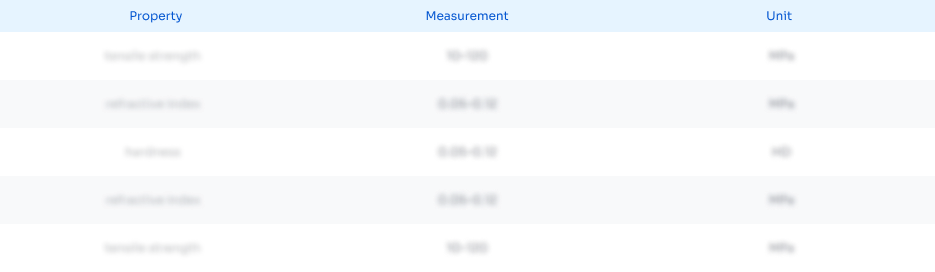
Abstract
Description
Claims
Application Information
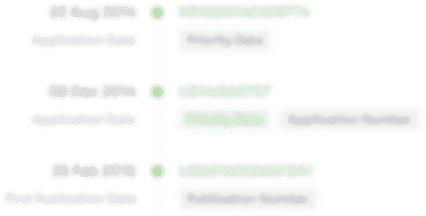
- R&D Engineer
- R&D Manager
- IP Professional
- Industry Leading Data Capabilities
- Powerful AI technology
- Patent DNA Extraction
Browse by: Latest US Patents, China's latest patents, Technical Efficacy Thesaurus, Application Domain, Technology Topic, Popular Technical Reports.
© 2024 PatSnap. All rights reserved.Legal|Privacy policy|Modern Slavery Act Transparency Statement|Sitemap|About US| Contact US: help@patsnap.com