Cross-modal retrieval method based on label fine-grained self-supervision
A cross-modal, fine-grained technology, applied in the field of cross-modal retrieval, can solve the problems of difficult measurement of cross-modal similarity, different modal distribution and inconsistent representation, etc.
- Summary
- Abstract
- Description
- Claims
- Application Information
AI Technical Summary
Problems solved by technology
Method used
Image
Examples
Embodiment Construction
[0018] The technical solutions in the embodiments of the present invention will be clearly and completely described below in conjunction with the accompanying drawings in the embodiments of the present invention. Obviously, the described embodiments are only some, not all, embodiments of the present invention. Based on the embodiments of the present invention, all other embodiments obtained by persons of ordinary skill in the art without making creative efforts belong to the protection scope of the present invention.
[0019] Such as figure 1 As shown, it is a flowchart of a cross-modal retrieval method based on label fine-grained self-supervision according to an embodiment of the present invention. The method includes the following steps:
[0020] S101: Construct feature extraction network
[0021] For the two modalities in the database, a feature extraction network is constructed respectively, and a label semantic extraction network is constructed for labels. Extract the i...
PUM
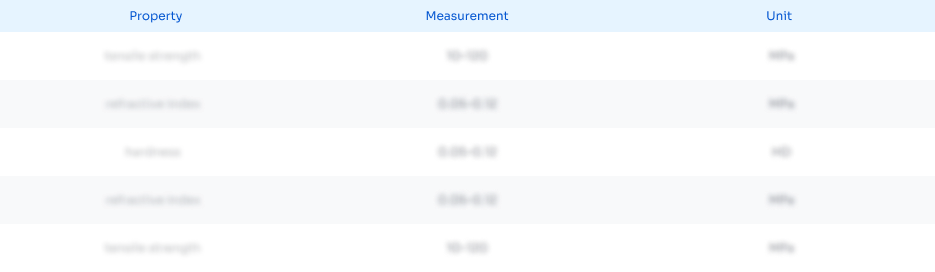
Abstract
Description
Claims
Application Information
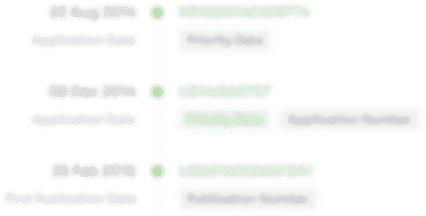
- R&D
- Intellectual Property
- Life Sciences
- Materials
- Tech Scout
- Unparalleled Data Quality
- Higher Quality Content
- 60% Fewer Hallucinations
Browse by: Latest US Patents, China's latest patents, Technical Efficacy Thesaurus, Application Domain, Technology Topic, Popular Technical Reports.
© 2025 PatSnap. All rights reserved.Legal|Privacy policy|Modern Slavery Act Transparency Statement|Sitemap|About US| Contact US: help@patsnap.com