Load monitoring using machine learning
A processor, energy technology for utility metering equipment that solves problems such as the difficulty of successful decomposition and limited availability hindering progress
- Summary
- Abstract
- Description
- Claims
- Application Information
AI Technical Summary
Problems solved by technology
Method used
Image
Examples
Embodiment Construction
[0017] Embodiments perform non-intrusive load monitoring using a novel learning scheme. NILM and decomposition refer to taking total energy use at a source location (e.g., energy use at a household provided by an advanced metering infrastructure) as input and estimating one or more appliances, electric vehicles, and Energy usage of other devices for energy. Embodiments utilize trained machine learning models to predict the energy usage of target devices based on the overall energy usage at the source location. For example, the target device could be a white goods or electric vehicle, the source location could be a home, and the trained machine learning model could receive as input the energy usage of the home and predict the energy usage of the target device (e.g., included in the overall Energy usage of the target device in Energy usage of the home).
[0018] Embodiments use labeled energy usage data to train a machine learning model. For example, a machine learning model,...
PUM
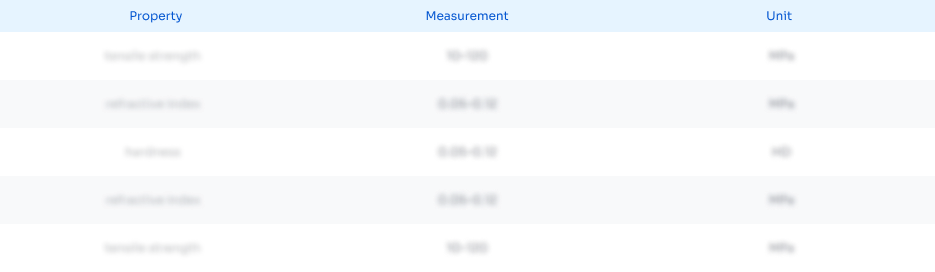
Abstract
Description
Claims
Application Information
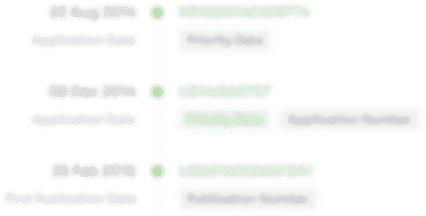
- R&D Engineer
- R&D Manager
- IP Professional
- Industry Leading Data Capabilities
- Powerful AI technology
- Patent DNA Extraction
Browse by: Latest US Patents, China's latest patents, Technical Efficacy Thesaurus, Application Domain, Technology Topic.
© 2024 PatSnap. All rights reserved.Legal|Privacy policy|Modern Slavery Act Transparency Statement|Sitemap