Method for a generative adversarial network with different hierarchical function combinations
A network and function technology, applied in the field of confrontation generation network with different levels of function combinations, can solve the problems of accuracy, generation efficiency, and no comprehensive consideration of features and gradients, so as to improve the poisoning effect, enhance the poisoning ability, and ensure the attack effect of effect
- Summary
- Abstract
- Description
- Claims
- Application Information
AI Technical Summary
Problems solved by technology
Method used
Image
Examples
Embodiment Construction
[0060] Since deep learning-based image recognition systems rely on the training of neural networks, which are vulnerable to adversarial attacks and cause misjudgment, the security of deep learning systems is vulnerable to carefully crafted adversarial examples. Researchers continue to propose new methods of adversarial attacks, but while neural networks are powerful enough to learn powerful models in the presence of natural noise, collecting data from untrusted sources makes neural networks vulnerable to successful attacks. The present invention proposes an adversarial generative network (DPGAN) method using different levels of function combinations, which destroys training data through data poisoning and ultimately destroys the entire learning process, thereby effectively improving the poisoning effect of poisoned samples.
[0061] In order to better understand the technical solution, the method of the present invention will be described in detail below in conjunction with the...
PUM
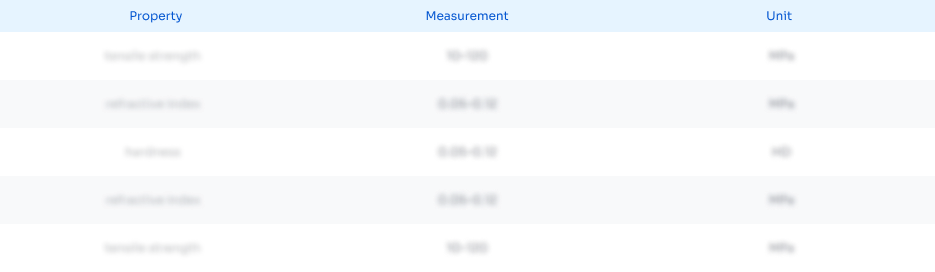
Abstract
Description
Claims
Application Information
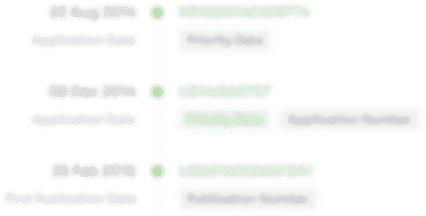
- Generate Ideas
- Intellectual Property
- Life Sciences
- Materials
- Tech Scout
- Unparalleled Data Quality
- Higher Quality Content
- 60% Fewer Hallucinations
Browse by: Latest US Patents, China's latest patents, Technical Efficacy Thesaurus, Application Domain, Technology Topic, Popular Technical Reports.
© 2025 PatSnap. All rights reserved.Legal|Privacy policy|Modern Slavery Act Transparency Statement|Sitemap|About US| Contact US: help@patsnap.com