Average error classification cost minimized classifier integrating method
A classifier and weak classifier technology, applied in the fields of instruments, character and pattern recognition, computer parts, etc., can solve problems such as difficulty in integrated learning methods
- Summary
- Abstract
- Description
- Claims
- Application Information
AI Technical Summary
Problems solved by technology
Method used
Image
Examples
Embodiment 1
[0130] Combine below figure 1 Describe the specific process steps of a classifier integration method for multi-category cost-sensitive learning provided by the present invention. The method includes the following steps:
[0131] S1. Obtain a training sample set S;
[0132] S2. Initialize sample weights and assign initial values, Among them, i=1,..., m, l=1,..., K, y i ∈ {1, 2, ..., K}, Z 0 for The normalization factor of c(y i , l) means y i The cost of class being wrongly divided into l class, m is the number of training samples;
[0133] S3. Iterate T times, train to obtain T best weak classifiers, and realize through steps S31-S33:
[0134] S31. Based on weighted value The training sample set S trains the weak classifier, t=1,...,T, through steps S311-S313 to achieve: S311, the division of the corresponding sample set S, calculate where j=1,...,n t , l represents the class in the multi-classification problem, x i represents the i-th sample, express The ...
Embodiment 2
[0145] Utilize the classifier integration method of multi-classification cost-sensitive learning of the present invention can realize a kind of multi-classification continuous AdaBoost integrated learning method, and its similarity with embodiment one is no longer repeated, and its difference is:
[0146] The method for assigning initial values to the training samples in step S2 is: i=1,...,m,l=1,...,K,Z 0 is a normalization factor, where c(i, i)=0, and c(i, j)=1 when i≠j. At this time, the average misclassification cost is simplified to the training error rate, and the classifier ensemble method for multi-classification cost-sensitive learning described in Embodiment 1 is simplified to a new multi-classification continuous AdaBoost ensemble learning method.
[0147] The method of the present invention introduces K weights to each sample, and when considering whether the target can be correctly classified, attention is paid to its opposite. equivalent to The l label su...
Embodiment 3
[0150] A kind of classifier ensemble method of multi-classification cost-sensitive learning proposed by the present invention and a kind of multi-classification continuous AdaBoost integrated learning method are used in practical application below, and integrate with the existing multi-classification continuous AdaBoost based on Bayesian statistical inference Compare learning methods.
[0151] The data selects the wine data set and random data set (Random data) on the UCI data set. The wine data has 3 types of labels, and the random data set is randomly generated. The random data used in the experiment uses the random matrix generation function rand(n) in MATLAB to generate an n×n matrix, intercepts the first d columns to obtain n samples with d attributes, and then divides the samples into 3 categories to obtain a random 3 Categorical datasets. The representativeness of the random data set is determined by the insignificant difference between the classes and the indistinct i...
PUM
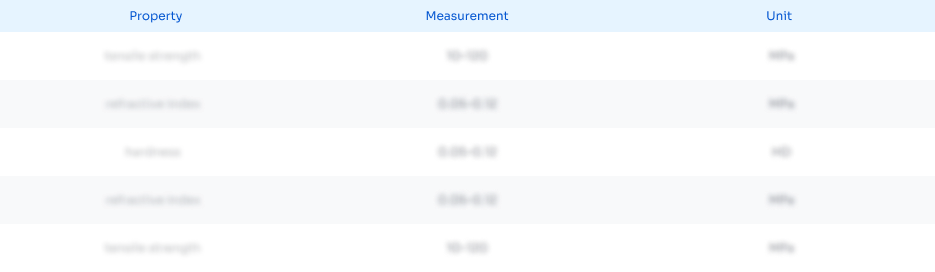
Abstract
Description
Claims
Application Information
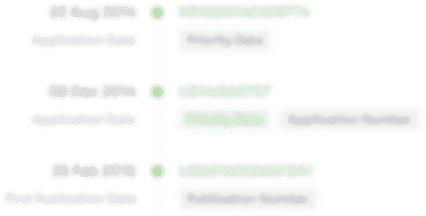
- R&D Engineer
- R&D Manager
- IP Professional
- Industry Leading Data Capabilities
- Powerful AI technology
- Patent DNA Extraction
Browse by: Latest US Patents, China's latest patents, Technical Efficacy Thesaurus, Application Domain, Technology Topic, Popular Technical Reports.
© 2024 PatSnap. All rights reserved.Legal|Privacy policy|Modern Slavery Act Transparency Statement|Sitemap|About US| Contact US: help@patsnap.com