Semantic segmentation network training method, image semantic segmentation method and devices
A technology of semantic segmentation and training images, applied in the field of computer vision, can solve the problems of low accuracy of semantic segmentation and poor training recognition effect, and achieve the effect of improving the training recognition effect.
- Summary
- Abstract
- Description
- Claims
- Application Information
AI Technical Summary
Problems solved by technology
Method used
Image
Examples
no. 1 example
[0029] Please refer to figure 2 , figure 2 A flow chart of the semantic segmentation network training method provided by the first embodiment of the present invention is shown. The semantic segmentation network training method includes the following steps:
[0030] Step S101, acquiring images to be trained.
[0031] In the embodiment of the present invention, the image to be trained may be a picture downloaded by the user through the network, or a picture taken by a camera or other shooting device. The images to be trained include multiple objects of different sizes, for example, people, sky, vehicles, animals, trees, and so on.
[0032]In the embodiment of the present invention, while obtaining the image to be trained, it is also necessary to obtain the original label map of the image to be trained. The original label map is information provided in advance, which includes object category information, that is, the original label map annotation The object category to whic...
Embodiment approach
[0042] As an implementation, the processing process of the mask convolution feature extraction sub-network can be as follows: First, input the pre-extraction feature map containing the blocks and overall features of the image to be trained into the convolution layer and the Argmax layer in sequence to generate the pre-extraction The recognition map, the pre-extraction recognition map is the object category map marked according to the pre-extraction feature map, that is to say, the pre-extraction recognition map is marked with the object category to which each pixel in the pre-extraction feature map belongs; then, using the traditional feature extraction The pooling layer in the sub-network performs scale down processing on the pre-extracted feature map to obtain the downsampled feature map, and then the downsampled feature map is input into the convolutional layer, Argmax layer and upsampling layer in turn to generate the downsampled recognition map , the recognition map after ...
no. 2 example
[0086] Please refer to Figure 7 , Figure 7 A flow chart of the image semantic segmentation method provided by the second embodiment of the present invention is shown. The image semantic segmentation method includes the following steps:
[0087] Step S201, acquiring an original image to be segmented.
[0088] In the embodiment of the present invention, the original image to be segmented may be an image that requires image semantic segmentation, which may be a photo taken by a camera or other shooting device.
[0089] Step S202, input the original image into the semantic segmentation network trained by using the semantic segmentation model training method of the first embodiment, and obtain the semantic segmentation result of the original image.
[0090] In the embodiment of the present invention, the semantic segmentation result of the original image includes the object category to which each pixel in the original image belongs.
PUM
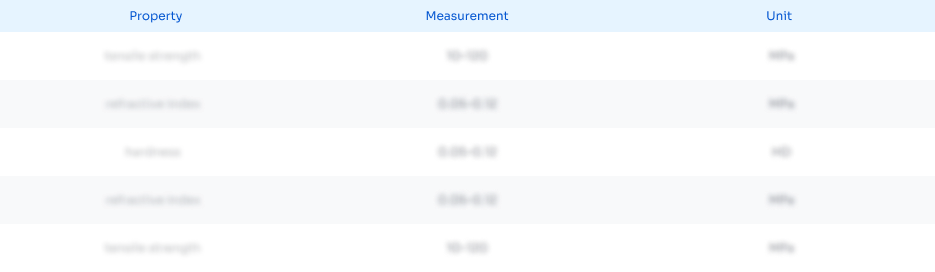
Abstract
Description
Claims
Application Information
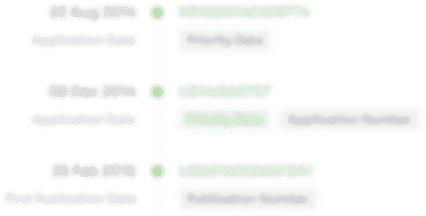
- R&D
- Intellectual Property
- Life Sciences
- Materials
- Tech Scout
- Unparalleled Data Quality
- Higher Quality Content
- 60% Fewer Hallucinations
Browse by: Latest US Patents, China's latest patents, Technical Efficacy Thesaurus, Application Domain, Technology Topic, Popular Technical Reports.
© 2025 PatSnap. All rights reserved.Legal|Privacy policy|Modern Slavery Act Transparency Statement|Sitemap|About US| Contact US: help@patsnap.com