Convolutional neural network adversarial transfer learning method based on Waserstein distance and application thereof
A convolutional neural network and transfer learning technology, applied in the field of industrial process fault diagnosis, can solve problems such as difficult application and high requirements for neural network structure, improve judgment accuracy, high accuracy, and solve the waste of computing resources and training time Effect
- Summary
- Abstract
- Description
- Claims
- Application Information
AI Technical Summary
Problems solved by technology
Method used
Image
Examples
Embodiment 1
[0048] A Convolutional Neural Network Adversarial Migration Learning Method 100 Based on Wasserstein Distance, Such as figure 1 shown, including:
[0049] Step 110, from the source domain labeled data set and the target domain data set, determine the source domain labeled sample set and the target domain sample set;
[0050] Step 120, using the convolutional neural network to be transferred to learn, obtain the source domain feature set and source domain fault judgment set of the source domain labeled sample set and the target feature set of the target domain sample set;
[0051] Step 130, with the goal of maximizing the Wasserstein distance between the source domain feature set and the target feature set and minimizing the sum of the Wasserstein distance and the judgment loss value of the source domain fault judgment set, adjusting the parameters of the convolutional neural network, based on the convergence Criterion, repeat step 110, or complete the adversarial transfer lea...
Embodiment 2
[0083] A convolutional neural network for industrial process fault diagnosis, obtained by training with any Wasserstein distance-based convolutional neural network confrontation transfer learning method as described in the first embodiment.
[0084] Based on the correlation between tasks, the convolutional neural network obtained by using any of the above-mentioned convolutional neural network adversarial transfer learning methods based on the Wasserstein distance can be used to learn the deep adversarial transfer learning of the convolutional neural network corresponding to the source domain. The convolutional neural network with high precision in the source domain and the target domain solves to a certain extent the waste of computing resources and training time caused by the need to rebuild the deep learning model from scratch in the face of new fault diagnosis tasks in the existing technology and the lack of Technical issues with sufficient sample data in the target domain....
Embodiment 3
[0086] An industrial process fault diagnosis method, based on any industrial process fault diagnosis convolutional neural network as described in the second embodiment above, when any convolutional neural network based on the Wasserstein distance described in the first embodiment above is received against When transferring a new sample of the target domain in the learning method, the industrial process fault judgment result corresponding to the new sample is obtained.
[0087] The convolutional neural network trained by any of the above-mentioned Wasserstein distance-based convolutional neural networks against transfer learning methods is used for industrial process fault diagnosis. On the premise of ensuring safety and high efficiency, the fault diagnosis accuracy is higher.
[0088] In order to verify the effectiveness of the above-mentioned deep adversarial transfer learning on the problem of fault diagnosis, this example introduces the benchmark bearing fault data set obtai...
PUM
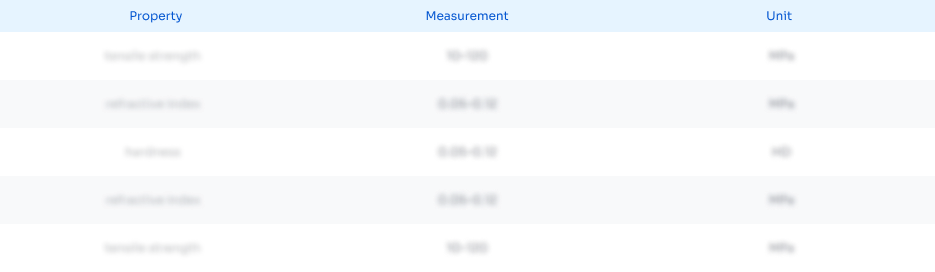
Abstract
Description
Claims
Application Information
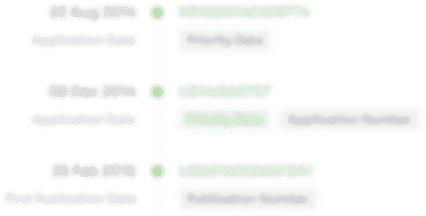
- R&D
- Intellectual Property
- Life Sciences
- Materials
- Tech Scout
- Unparalleled Data Quality
- Higher Quality Content
- 60% Fewer Hallucinations
Browse by: Latest US Patents, China's latest patents, Technical Efficacy Thesaurus, Application Domain, Technology Topic, Popular Technical Reports.
© 2025 PatSnap. All rights reserved.Legal|Privacy policy|Modern Slavery Act Transparency Statement|Sitemap|About US| Contact US: help@patsnap.com