Privacy-preserving machine learning
A machine learning and privacy protection technology, applied in the fields of digital data protection, neural learning methods, instruments, etc., can solve problems such as data that cannot be used for inference
- Summary
- Abstract
- Description
- Claims
- Application Information
AI Technical Summary
Problems solved by technology
Method used
Image
Examples
Embodiment approach
[0289] The system is implemented in C++. In all our experiments, the domain size was set to 2 64 . Thus, we observe that modulo arithmetic can be implemented in C++ using regular arithmetic on unsigned long integer types at no additional cost. This is significantly faster than any number theory library capable of handling operations in arbitrary domains. For example, we test that integer addition (multiplication) is 100× faster than modular addition (multiplication) in the same domain implemented in the GMP [5] or NTL [7] libraries. More generally, the finite field Any element in can be represented by one or several unsigned long integers, and addition (multiplication) can be calculated by one or several regular additions (multiplications) plus some bit operations. The speedup of such implementations is similar to using general number theory libraries. We use the Eigen library [2] to handle matrix operations. OT and obfuscation circuits are implemented using the EMP...
PUM
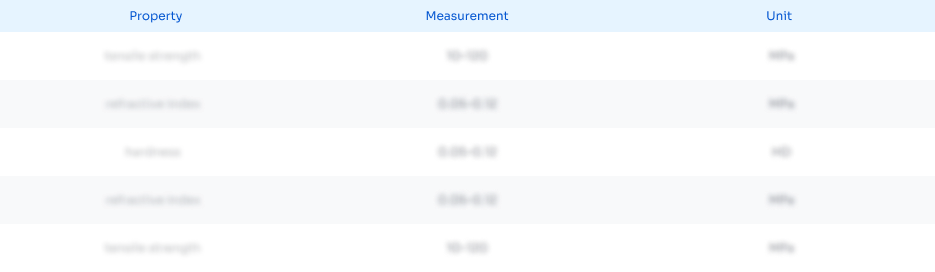
Abstract
Description
Claims
Application Information
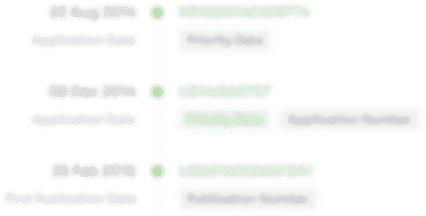
- R&D Engineer
- R&D Manager
- IP Professional
- Industry Leading Data Capabilities
- Powerful AI technology
- Patent DNA Extraction
Browse by: Latest US Patents, China's latest patents, Technical Efficacy Thesaurus, Application Domain, Technology Topic, Popular Technical Reports.
© 2024 PatSnap. All rights reserved.Legal|Privacy policy|Modern Slavery Act Transparency Statement|Sitemap|About US| Contact US: help@patsnap.com