An Unsupervised Domain Adaptation Method Based on Adversarial Learning Loss Function
A technique of loss function, adaptation method, applied in the field of unsupervised domain adaptation of transfer learning, which can solve problems such as performance degradation and no theoretical guarantees
- Summary
- Abstract
- Description
- Claims
- Application Information
AI Technical Summary
Problems solved by technology
Method used
Image
Examples
Embodiment Construction
[0066] The present invention will be further described in detail below with reference to the accompanying drawings and embodiments. It should be pointed out that the following embodiments are intended to facilitate the understanding of the present invention, but do not have any limiting effect on it.
[0067] like figure 1 and figure 2 As shown, an unsupervised domain adaptation method based on adversarial learning loss function, the framework of the present invention is mainly divided into two branches to deal with images of two domains respectively (see figure 2 ): (1) (dotted line) The source domain image passes through the feature extraction network G to generate high-level features, and passes through the classifier C and the real label for cross-entropy loss. Label. (2) (solid line) The target domain image passes through the feature extraction network G to generate high-level features, and passes through the classifier C to generate pseudo-labels. On the other hand, ...
PUM
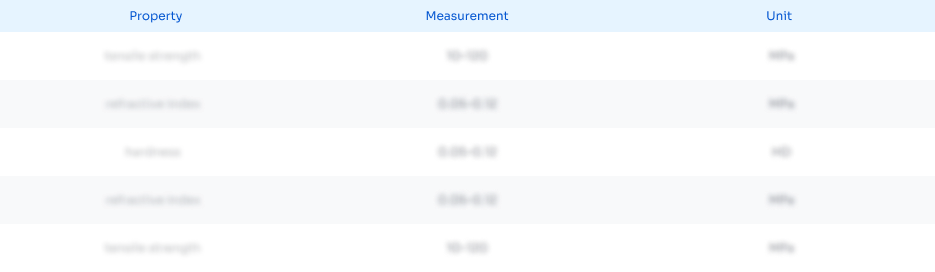
Abstract
Description
Claims
Application Information
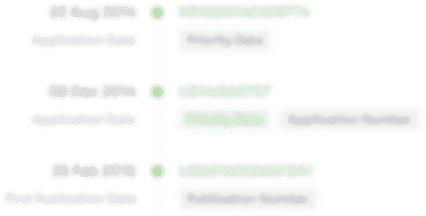
- R&D Engineer
- R&D Manager
- IP Professional
- Industry Leading Data Capabilities
- Powerful AI technology
- Patent DNA Extraction
Browse by: Latest US Patents, China's latest patents, Technical Efficacy Thesaurus, Application Domain, Technology Topic, Popular Technical Reports.
© 2024 PatSnap. All rights reserved.Legal|Privacy policy|Modern Slavery Act Transparency Statement|Sitemap|About US| Contact US: help@patsnap.com