Traffic violation severity level prediction method based on Bayesian network
A technology of Bayesian network, forecasting method, applied in the field of traffic psychology, to achieve the effect of good severity level
- Summary
- Abstract
- Description
- Claims
- Application Information
AI Technical Summary
Problems solved by technology
Method used
Image
Examples
Embodiment 1
[0036] A method for predicting the severity level of traffic violations based on Bayesian networks, comprising the following steps:
[0037] S1: Determine the variables of the Bayesian model;
[0038] S2: Establish a Bayesian model and train the model.
[0039] Further, the specific process of the step S1 is:
[0040] According to the analysis of the driver's gender, age, driving experience, time period of illegal incidents, types of illegal vehicles, vehicle attribution and severity of traffic violations, these factors are determined as variables of the Bayesian model, a training set and parameters in the training set can be expressed as figure 1 Shown:
[0041] X={G,A,D,T,W,V,O,L} (1)
[0042] in:
[0043] G={0,1} means gender, 0 means female, 1 means male;
[0044] A={0,1,2,3,4,5} means age, where 0 means 18-20 years old, 1 means 21-30 years old, 2 means 31-40 years old, 3 means 41-50 years old, 4 means 51 years old -60 years old, 5 means 61 years old and above;
[...
PUM
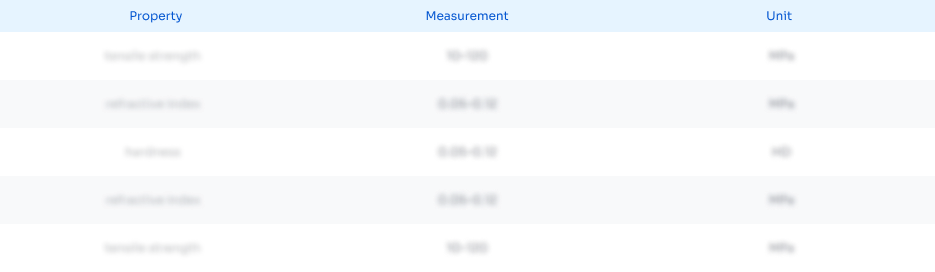
Abstract
Description
Claims
Application Information
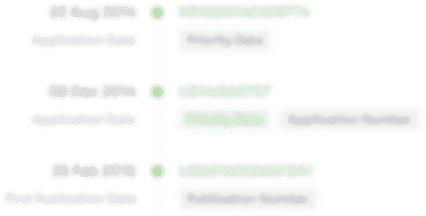
- R&D Engineer
- R&D Manager
- IP Professional
- Industry Leading Data Capabilities
- Powerful AI technology
- Patent DNA Extraction
Browse by: Latest US Patents, China's latest patents, Technical Efficacy Thesaurus, Application Domain, Technology Topic, Popular Technical Reports.
© 2024 PatSnap. All rights reserved.Legal|Privacy policy|Modern Slavery Act Transparency Statement|Sitemap|About US| Contact US: help@patsnap.com