LSTM (Long Short-Term Memory) based time sequence network anomaly detection method and device
A time series and network anomaly technology, applied in the field of information security, can solve the problems of the traditional network anomaly detection application being in the early stage of exploration, and achieve the effect of intuitive and obvious distinction, complete development and fitting, and good effect.
- Summary
- Abstract
- Description
- Claims
- Application Information
AI Technical Summary
Problems solved by technology
Method used
Image
Examples
Embodiment 1
[0065] The embodiment of the present invention provides an LSTM-based time series network anomaly detection method, which is applied to the server, see figure 1 As shown, the method includes the following steps:
[0066] S101: Obtain an actual measured value of network traffic to be detected.
[0067] During specific implementation, firstly, the server obtains the actual measurement value of the network traffic to be detected.
[0068] S102: Input the actual measured value of the network traffic to be detected into the LSTM-based time series network traffic prediction model to obtain the predicted value of the network traffic to be detected.
[0069] Input the actual measurement value of the network traffic to be detected obtained above into the pre-trained model to obtain the predicted value of the network traffic to be detected. The model is an LSTM-based time series network anomaly detection model, which specifically includes: RNN cycle Neural network and valve nodes of e...
Embodiment 2
[0113] An embodiment of the present invention provides an LSTM-based time series network anomaly detection device, see Figure 7 As shown, the device includes: a first data acquisition module 51 , a model prediction module 52 , and an anomaly detection module 53 .
[0114] Among them, the first data acquisition module 51 is used to obtain the actual measured value of the network traffic to be detected; the model prediction module 52 is used to input the actual measured value of the network traffic to be detected into the time series network traffic prediction model based on LSTM, and obtain The predicted value of the network traffic to be detected; the abnormal detection module 53 is used to compare the actual measurement value of the network traffic to be detected with the predicted value of the network traffic to be detected, and obtain the abnormal data detection result of the network traffic to be detected.
[0115] In addition, it also includes: a second data acquisition ...
PUM
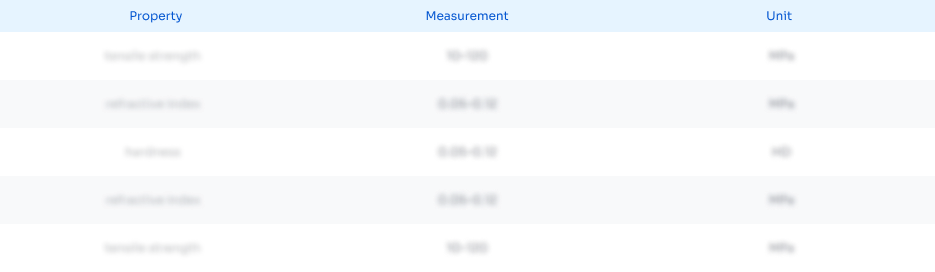
Abstract
Description
Claims
Application Information
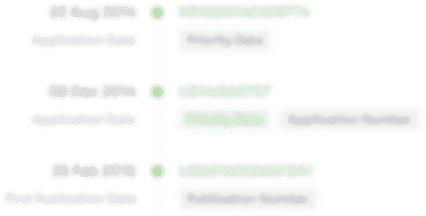
- R&D Engineer
- R&D Manager
- IP Professional
- Industry Leading Data Capabilities
- Powerful AI technology
- Patent DNA Extraction
Browse by: Latest US Patents, China's latest patents, Technical Efficacy Thesaurus, Application Domain, Technology Topic.
© 2024 PatSnap. All rights reserved.Legal|Privacy policy|Modern Slavery Act Transparency Statement|Sitemap