Malicious code multi-model intersection detection method based on statistical learning
A malicious code and statistical learning technology, applied in computing, computer security devices, instruments, etc., can solve the problems of malicious code variation, machine learning model prediction results that cannot be compared with each other and common defense, etc. Effect
- Summary
- Abstract
- Description
- Claims
- Application Information
AI Technical Summary
Problems solved by technology
Method used
Image
Examples
Embodiment Construction
[0052] The present invention takes the detection of botnets as an example for specific description, and any machine learning algorithm using threshold value comparison can be applied to the method as a bottom-level scoring function.
[0053] 1. Malicious behavior on the Internet
[0054] In this embodiment, a data set including real network communication traffic is used, and the data set includes various botnets.
[0055] 2. Extract features
[0056] In this embodiment, multiple features related to each network trace are extracted. These characteristics include communication frequency, communication duration, number of bytes sent and received, number of packets sent and received, protocol type, and proportion of using 3 ports (SystemPorts, UserPorts, Dynamic or Private Ports), etc., and are not limited Based on these features, the network trace is abstracted into a feature vector. The format of the vector is as Figure 8 As shown, each column is a feature point, and each r...
PUM
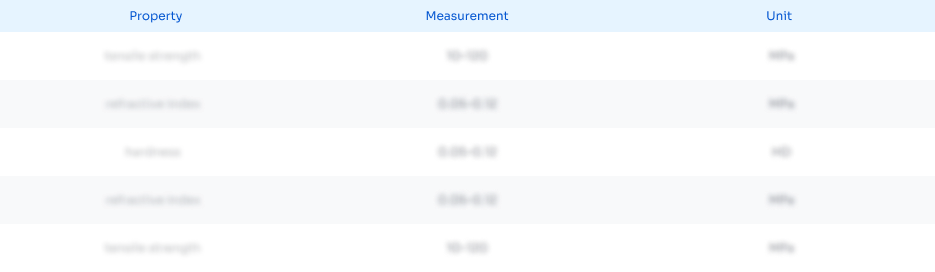
Abstract
Description
Claims
Application Information
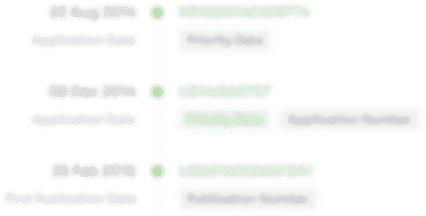
- R&D Engineer
- R&D Manager
- IP Professional
- Industry Leading Data Capabilities
- Powerful AI technology
- Patent DNA Extraction
Browse by: Latest US Patents, China's latest patents, Technical Efficacy Thesaurus, Application Domain, Technology Topic, Popular Technical Reports.
© 2024 PatSnap. All rights reserved.Legal|Privacy policy|Modern Slavery Act Transparency Statement|Sitemap|About US| Contact US: help@patsnap.com