Multi-model malicious code detection method based on reliability probability interval
A classification method and algorithm technology, applied in character and pattern recognition, instrument, platform integrity maintenance, etc., can solve problems such as concept drift, and achieve the effect of coping with variation and improving variation
- Summary
- Abstract
- Description
- Claims
- Application Information
AI Technical Summary
Problems solved by technology
Method used
Image
Examples
Embodiment Construction
[0046] The present invention takes the detection of botnets as an example for specific description, and the underlying scoring functions take KNN and KDE algorithms as examples, and any machine learning algorithm that uses threshold comparison can be applied to the method as a scoring function.
[0047] 1. Malicious behavior on the Internet
[0048] In this embodiment, a data set containing real network communication traffic is used, and the data set includes three types of botnets: IRC, HTTP, and P2P.
[0049] IRC botnet dataset (sample):
[0050]
[0051] HTTP botnet dataset (sample):
[0052]
[0053] P2P botnet dataset (sample):
[0054]
[0055] 2. Extract network behavior features
[0056] In this embodiment, 10 features related to each network trace are extracted. These characteristics include communication frequency, communication duration, number of bytes sent and received, number of packets sent and received, protocol type and proportion of using 3 ports...
PUM
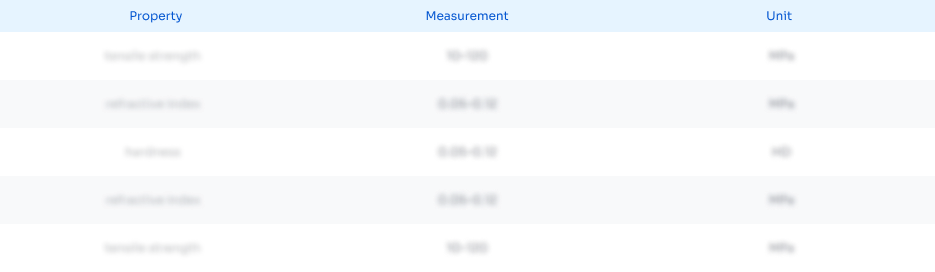
Abstract
Description
Claims
Application Information
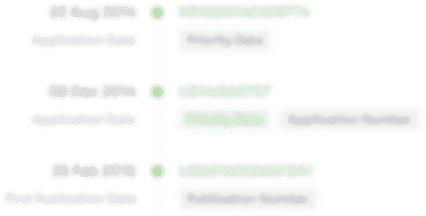
- R&D Engineer
- R&D Manager
- IP Professional
- Industry Leading Data Capabilities
- Powerful AI technology
- Patent DNA Extraction
Browse by: Latest US Patents, China's latest patents, Technical Efficacy Thesaurus, Application Domain, Technology Topic, Popular Technical Reports.
© 2024 PatSnap. All rights reserved.Legal|Privacy policy|Modern Slavery Act Transparency Statement|Sitemap|About US| Contact US: help@patsnap.com