A segnet remote sensing image semantic segmentation method combined with random walk
A random walk and remote sensing image technology, applied in the field of information, can solve the problems of edge recognition and positioning errors of ground objects, edge segmentation is not smooth enough, and there are many plaque noises, etc., to achieve accurate edge positioning, optimize segmentation results, and improve output quality Effect
- Summary
- Abstract
- Description
- Claims
- Application Information
AI Technical Summary
Problems solved by technology
Method used
Image
Examples
Embodiment Construction
[0030] The present invention will be described in detail below in conjunction with the accompanying drawings and embodiments.
[0031] Such as figure 1 Shown, the present invention is concretely realized as follows:
[0032] (1) SegNet initial segmentation
[0033] In the traditional SegNet image detection, compared with the center area, the area near the edge of the detected image has less information available for classification, and the segmentation accuracy is lower than that of the window center area.
[0034] The present invention adopts a method of performing multiple predictions on the same position pixel in combination with multiple detection windows. Use a window of the same size as the input image to perform sliding sampling on the image, and the obtained sampled image is a window image, input the window image to SegNet, and output the predicted category and category intensity information corresponding to each original window image pixel, that is, pixel-by-pixel p...
PUM
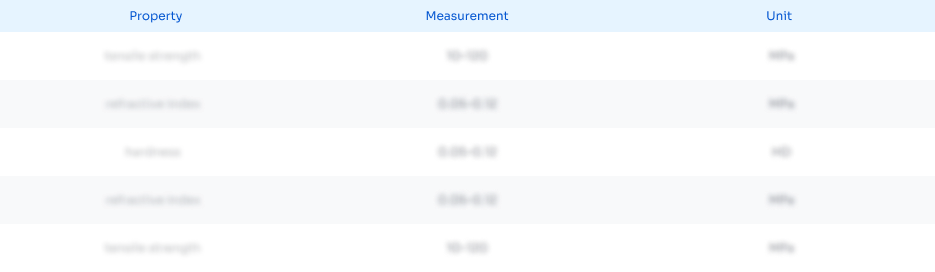
Abstract
Description
Claims
Application Information
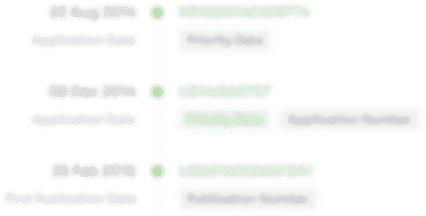
- Generate Ideas
- Intellectual Property
- Life Sciences
- Materials
- Tech Scout
- Unparalleled Data Quality
- Higher Quality Content
- 60% Fewer Hallucinations
Browse by: Latest US Patents, China's latest patents, Technical Efficacy Thesaurus, Application Domain, Technology Topic, Popular Technical Reports.
© 2025 PatSnap. All rights reserved.Legal|Privacy policy|Modern Slavery Act Transparency Statement|Sitemap|About US| Contact US: help@patsnap.com