Method for constructing target detection adaptive model based on CycleGAN and pseudo tag
An adaptive model and target detection technology, applied in the field of deep learning, can solve problems such as target detection domain drift
- Summary
- Abstract
- Description
- Claims
- Application Information
AI Technical Summary
Problems solved by technology
Method used
Image
Examples
Embodiment Construction
[0055] The characteristics and performance of the present invention will be described in further detail below in conjunction with the examples.
[0056] Such as figure 1 As shown, a method for building an adaptive model for target detection based on CycleGAN and pseudo-labels in this embodiment includes:
[0057] S1, source domain dataset and target domain dataset preprocessing, use the preprocessed source domain dataset and target domain dataset to execute S2-S3;
[0058] S11, source domain dataset preprocessing:
[0059] The source domain dataset X containing labeled data S ={(s 1 ,q 1 , a 1 ), (s 2 ,q 2 , a 2 ),..., (s n ,q n , a n )} to perform size normalization operation to obtain the preprocessed source domain dataset Among them, n is X S The number of image samples in , s j stands for x S In the jth image sample, q j stands for x S The label data contained in the jth image sample in a j stands for x S The position data contained in the jth image sam...
PUM
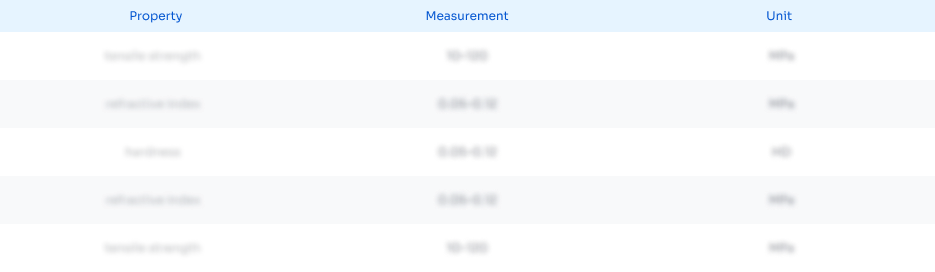
Abstract
Description
Claims
Application Information
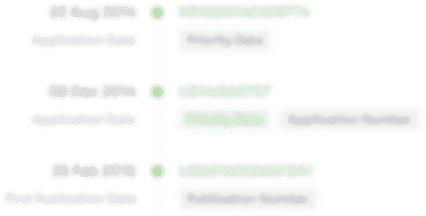
- Generate Ideas
- Intellectual Property
- Life Sciences
- Materials
- Tech Scout
- Unparalleled Data Quality
- Higher Quality Content
- 60% Fewer Hallucinations
Browse by: Latest US Patents, China's latest patents, Technical Efficacy Thesaurus, Application Domain, Technology Topic, Popular Technical Reports.
© 2025 PatSnap. All rights reserved.Legal|Privacy policy|Modern Slavery Act Transparency Statement|Sitemap|About US| Contact US: help@patsnap.com