Texture feature extraction method fused with visual significance and gray level co-occurrence matrix (GLCM)
A technology of gray co-occurrence moment and texture feature, applied in the field of computer vision, it can solve the problems of slow calculation speed, large information redundancy, and inability to describe the visual sensitivity of human eyes.
- Summary
- Abstract
- Description
- Claims
- Application Information
AI Technical Summary
Problems solved by technology
Method used
Image
Examples
Embodiment 1
[0068] Such as figure 1 As shown, the present invention is a texture feature extraction method that combines visual saliency and gray co-occurrence moments, and its steps are:
[0069] 1. Initialization;
[0070] Determine the target detection window, basic block, super block size (shown in step ①). The target detection window is determined based on the experience of detecting targets. For example, the detection window for pedestrians is 36*108, the basic block size is 9*12, and the super block size is 18*24. The parameters can be adjusted appropriately according to the size of the actual target;
[0071] If the image is successfully acquired, that is, the image file is successfully read or the camera captures the image successfully (step ②), continue preprocessing including filtering image noise (step ③) to provide more accurate input for the next step, otherwise end ( Step ⑧).
[0072] 2. Feature extraction;
[0073] Calculate the significance factor in units of basic b...
PUM
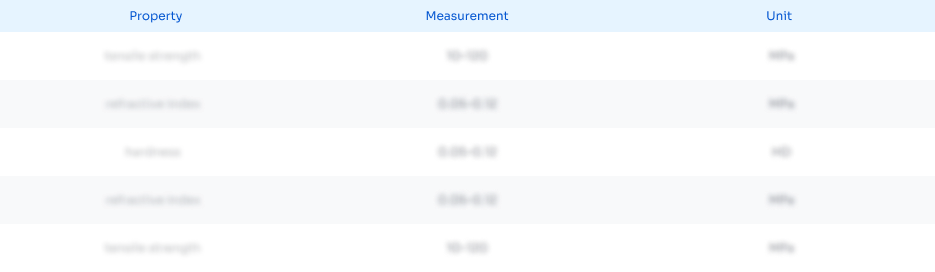
Abstract
Description
Claims
Application Information
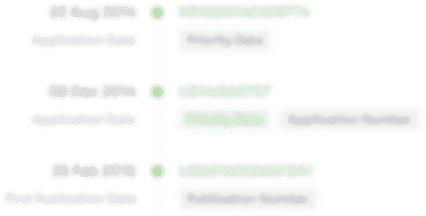
- R&D Engineer
- R&D Manager
- IP Professional
- Industry Leading Data Capabilities
- Powerful AI technology
- Patent DNA Extraction
Browse by: Latest US Patents, China's latest patents, Technical Efficacy Thesaurus, Application Domain, Technology Topic.
© 2024 PatSnap. All rights reserved.Legal|Privacy policy|Modern Slavery Act Transparency Statement|Sitemap