Zero sample learning method based on global semantic consistency network
A sample learning and consistent technology, applied in the field of machine learning, can solve problems such as the loss of absolute distance information in sorting, no upper bound on the fitness score, and no semantic structure learned in sorting, so as to achieve enhanced interpretability and strong interpretability , Ease of use
- Summary
- Abstract
- Description
- Claims
- Application Information
AI Technical Summary
Benefits of technology
Problems solved by technology
Method used
Image
Examples
Embodiment Construction
[0039] The specific implementation settings of the method of the present invention are given below, and the performance test and analysis of the method of the present invention are given to describe the implementation process of the method of the present invention in more detail.
[0040] 1. Method implementation
[0041] The available data for the zero-shot learning task is as follows: visible class images and their labels, and the attribute matrix W composed of the scores of all classes on each attribute. A simple and scalable implementation method is: put the given visible class image into the folder corresponding to the class label, and ensure that the seen class and unseen class folder numbers are consistent with the class label numbers during cross-entropy training. Save the number of the seen class and the unseen class, so that the network structure of training and testing is the same, no need to change, only need to output the highest probability class in the unseen cl...
PUM
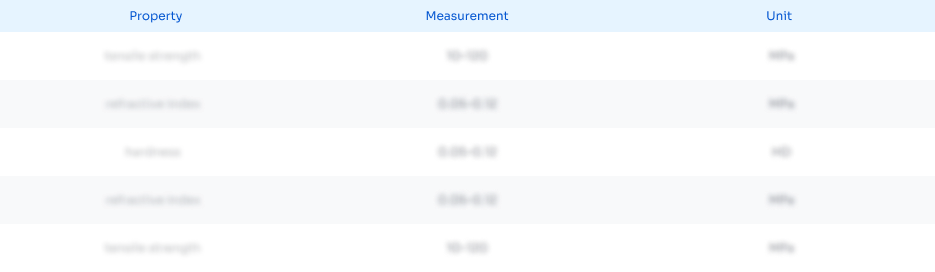
Abstract
Description
Claims
Application Information
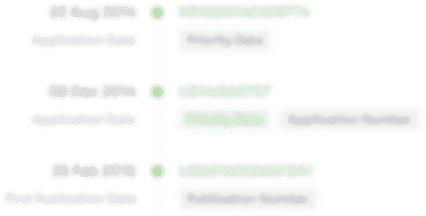
- R&D
- Intellectual Property
- Life Sciences
- Materials
- Tech Scout
- Unparalleled Data Quality
- Higher Quality Content
- 60% Fewer Hallucinations
Browse by: Latest US Patents, China's latest patents, Technical Efficacy Thesaurus, Application Domain, Technology Topic, Popular Technical Reports.
© 2025 PatSnap. All rights reserved.Legal|Privacy policy|Modern Slavery Act Transparency Statement|Sitemap|About US| Contact US: help@patsnap.com