Fault classification method based on one-dimensional multi-path convolutional neural network
A technology of convolutional neural network and fault classification, which is applied in the field of fault classification based on one-dimensional multi-channel convolutional neural network, can solve problems such as low accuracy and precision of fault identification, sensitive variable order, etc., and achieve the goal of overcoming the order of variables Effects of sensitivity, feature diversity, and high robustness
- Summary
- Abstract
- Description
- Claims
- Application Information
AI Technical Summary
Problems solved by technology
Method used
Image
Examples
Embodiment Construction
[0052]In order to make the object, technical solution and advantages of the present invention clearer, the present invention will be further described in detail below in conjunction with the accompanying drawings and embodiments. It should be understood that the specific embodiments described here are only used to explain the present invention, not to limit the present invention.
[0053] refer to figure 1 A fault classification method based on a one-dimensional multi-channel convolutional neural network model provided by an embodiment of the present invention includes the following steps:
[0054] (1) Collect data under various fault states in the industrial process, and perform fault marking and standardization processing on these data to construct a data set;
[0055] (2) Build a multi-channel one-dimensional convolutional neural network model, and perform feature extraction on the data set; the multi-channel one-dimensional convolutional neural network model includes sequ...
PUM
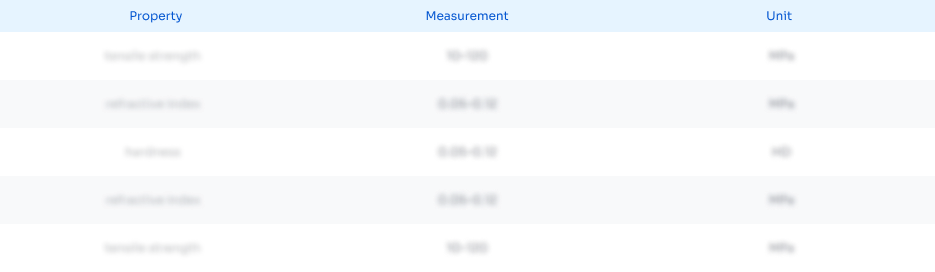
Abstract
Description
Claims
Application Information
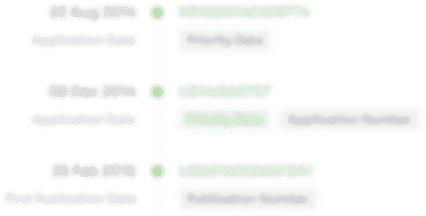
- Generate Ideas
- Intellectual Property
- Life Sciences
- Materials
- Tech Scout
- Unparalleled Data Quality
- Higher Quality Content
- 60% Fewer Hallucinations
Browse by: Latest US Patents, China's latest patents, Technical Efficacy Thesaurus, Application Domain, Technology Topic, Popular Technical Reports.
© 2025 PatSnap. All rights reserved.Legal|Privacy policy|Modern Slavery Act Transparency Statement|Sitemap|About US| Contact US: help@patsnap.com