A low-rank sparse representation image feature learning method based on Laplace regularization
An image feature and learning method technology, applied in the field of face image recognition, can solve the problems of poor stability, damage to the overall performance of the method, weak feature discrimination, etc., to achieve strong robustness, reduce time complexity, improve accuracy and The effect of robustness
- Summary
- Abstract
- Description
- Claims
- Application Information
AI Technical Summary
Problems solved by technology
Method used
Image
Examples
Embodiment Construction
[0036] The drawings are for illustrative purposes only, and should not be construed as limitations on this patent; in order to better illustrate this embodiment, some parts in the drawings will be omitted, enlarged or reduced, and do not represent the size of the actual product;
[0037] For those skilled in the art, it is understandable that some well-known structures and descriptions thereof may be omitted in the drawings. The technical solutions of the present invention will be further described below in conjunction with the accompanying drawings and embodiments.
[0038] The ORL face database consists of a series of face images taken by the Olivetti Laboratory in Cambridge, England, from April 1992 to April 1994, with a total of 40 subjects of different ages, genders and races. There are 10 images for each person, a total of 400 grayscale images, and the resolution of each image is 32×32. This embodiment combines figure 1 Do a further detailed description.
[0039] A lo...
PUM
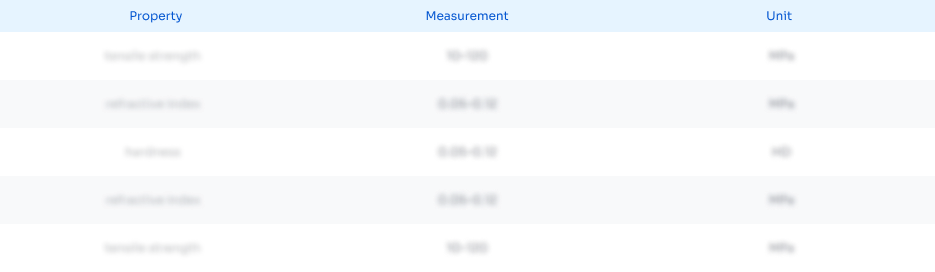
Abstract
Description
Claims
Application Information
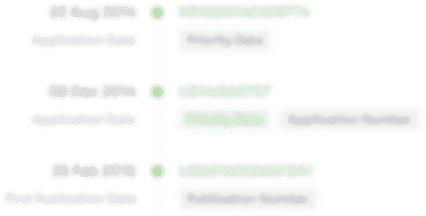
- R&D Engineer
- R&D Manager
- IP Professional
- Industry Leading Data Capabilities
- Powerful AI technology
- Patent DNA Extraction
Browse by: Latest US Patents, China's latest patents, Technical Efficacy Thesaurus, Application Domain, Technology Topic, Popular Technical Reports.
© 2024 PatSnap. All rights reserved.Legal|Privacy policy|Modern Slavery Act Transparency Statement|Sitemap|About US| Contact US: help@patsnap.com