A Song Clustering Method Based on Iterative K-means Algorithm
A clustering method and song technology, applied in the field of data processing, can solve the problems of low accuracy, low efficiency, and inability to search for similarity of songs by manual labeling, and achieve the effect of solving the difficulty of manual classification and solving the problem of accuracy rate disclosure
- Summary
- Abstract
- Description
- Claims
- Application Information
AI Technical Summary
Problems solved by technology
Method used
Image
Examples
Embodiment 1
[0060] A kind of song clustering method based on iterative k-means algorithm of the present embodiment, such as Figure 1-4 As shown, it specifically includes the following steps:
[0061] Step S1: Extract the Mel-frequency cepstral coefficients of the songs in the music library by time intervals, and obtain the MFCC vectors of each frame of each time period of each song;
[0062] Step S2: Carry out song ID identification on all MFCC vectors in step S1, and compile the song information to which each MFCC vector belongs into a data set;
[0063] Step S3: use the data set in step S2 as the input data to carry out k-means clustering for the first time, obtain the cluster information to which each MFCC vector belongs, and obtain the clustering result of the MFCC vector;
[0064] Step S4: Generate a K-dimensional label vector set corresponding to the song by the ratio of the MFCC vector contained in each song in each cluster; each song has and only one corresponding K-dimensional ...
Embodiment 2
[0068] This embodiment is based on Embodiment 1, and limits the Mel-frequency cepstral coefficient of the song in the music storehouse to be extracted by time division in the step S1 and obtains the 26-dimensional MFCC vector of each frame of each time period of each song, that is, the first time The feature vector of k-means clustering is a 26-dimensional MFCC vector. At the same time, in the step S3, the value of the total number of clusters K of the first k-means clustering is set to 50, that is, 50 MFCC vectors with iconic features in the music library are extracted through the first k-means clustering Represents a vector.
[0069] Based on the above limitations, a song clustering method based on the iterative k-means algorithm in this embodiment specifically includes steps S1-S5.
[0070] Step S1: Extract the Mel-frequency cepstrum coefficients of the songs in the music library by time intervals, and obtain the MFCC vectors of each frame of each time period of each song....
Embodiment 3
[0148] This embodiment is further optimized on the basis of Embodiment 1 and Embodiment 2. The beginning period of the song refers to the beginning of the song for 0-15 seconds, the climax period of the song refers to the beginning of the song climax for 0-20 seconds, and the end period of the song refers to The first 15 seconds of the last 20 seconds of the song.
[0149] That is to say, in the described step S1-1, all songs in the music library are preprocessed, and the beginning (first 15 seconds) of the song is extracted, the climax (the middle 20 seconds), and the ending (the first 15 seconds in the ending 20 seconds) generate three A WAV format file, as a representative part of each song, and carry out song ID identification on it.
[0150] Other parts of this embodiment are the same as those of Embodiment 1 or Embodiment 2, so details are not repeated here.
PUM
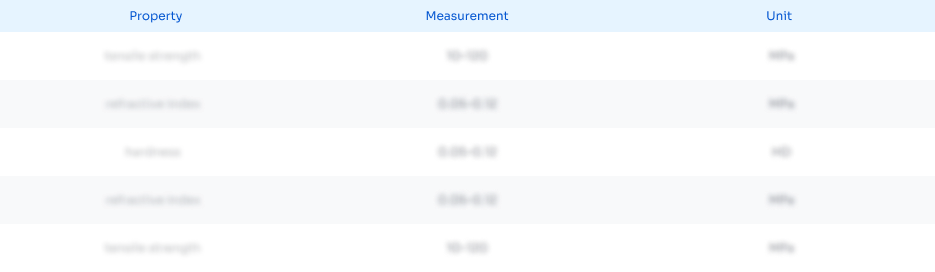
Abstract
Description
Claims
Application Information
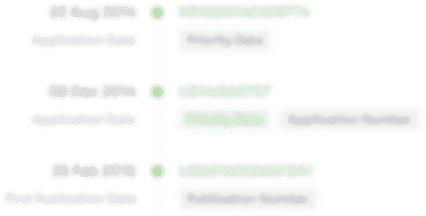
- R&D Engineer
- R&D Manager
- IP Professional
- Industry Leading Data Capabilities
- Powerful AI technology
- Patent DNA Extraction
Browse by: Latest US Patents, China's latest patents, Technical Efficacy Thesaurus, Application Domain, Technology Topic, Popular Technical Reports.
© 2024 PatSnap. All rights reserved.Legal|Privacy policy|Modern Slavery Act Transparency Statement|Sitemap|About US| Contact US: help@patsnap.com