A method for learning super-resolution network based on sub-band of cluster structure in wavelet domain
A super-resolution and wavelet domain technology, applied in the field of computer vision image super-resolution restoration, can solve the problems of blurred high-resolution images, low peak signal-to-noise ratio and image similarity structure index, and difficulty in restoring the details of a single high-definition image and other problems, to achieve good quantitative indicators and solve the effect of super-resolution recovery
- Summary
- Abstract
- Description
- Claims
- Application Information
AI Technical Summary
Problems solved by technology
Method used
Image
Examples
Embodiment Construction
[0064] Below in conjunction with accompanying drawing, further describe the present invention through embodiment, but do not limit the scope of the present invention in any way.
[0065] The present invention provides a method for jointly learning a super-resolution network based on group structure subbands in the wavelet domain, and constructs a novel super-resolution network: a group-type super-resolution image network; a group-type super-resolution image network includes two parts: a feature embedding network ( FEN) and Image Reconstruction Network (IRN).
[0066] (1) The overall structure of the network
[0067] Such as figure 1 As shown, our clique super-resolution image network mainly consists of two subnetworks: Feature Embedding Network (FEN) and Image Restoration Network (IRN). A feature embedding network represents a low-resolution input image as a set of feature maps. Note that the feature embedding network does not change the input image size (h, w), where h and...
PUM
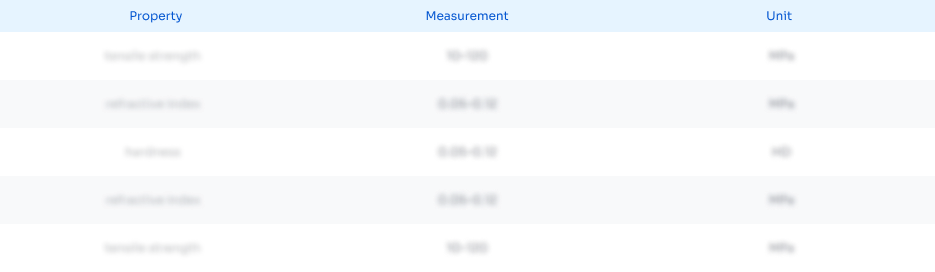
Abstract
Description
Claims
Application Information
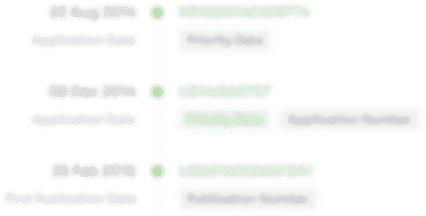
- Generate Ideas
- Intellectual Property
- Life Sciences
- Materials
- Tech Scout
- Unparalleled Data Quality
- Higher Quality Content
- 60% Fewer Hallucinations
Browse by: Latest US Patents, China's latest patents, Technical Efficacy Thesaurus, Application Domain, Technology Topic, Popular Technical Reports.
© 2025 PatSnap. All rights reserved.Legal|Privacy policy|Modern Slavery Act Transparency Statement|Sitemap|About US| Contact US: help@patsnap.com