Deep learning image restoration method under low sampling rate
A technology of deep learning and low sampling rate, applied in the field of image processing, can solve the problem of losing high-frequency content, achieve the effect of improving visual effects and reconstruction speed, improving quantitative indicators and reconstruction effects, and saving computing resources
- Summary
- Abstract
- Description
- Claims
- Application Information
AI Technical Summary
Problems solved by technology
Method used
Image
Examples
Embodiment Construction
[0023] The present invention provides a deep learning image restoration method at a low sampling rate. On the basis of AMP-NET, discrete wavelet transform is introduced. At the same time, the sampling matrix is processed by half tensor product, and the original image is decomposed into multi-scale , and then block-sample images of all scales, and reconstruct the original image, and finally get an image with better reconstruction quality than the previously proposed restoration algorithm. At this time, the network is called AMP-NET+, and the network model is applied to the image reconstruction process, which improves the image reconstruction quality and significantly improves the running speed.
[0024] In order to enable those skilled in the art to better understand the technical solutions of the present invention, the present invention will be further described in detail below in conjunction with the accompanying drawings and embodiments.
[0025] First, the semi-tensor com...
PUM
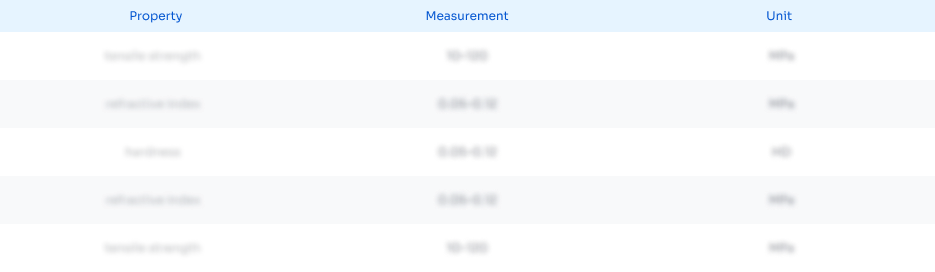
Abstract
Description
Claims
Application Information
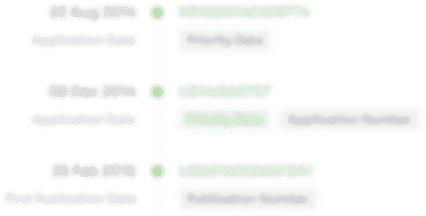
- R&D Engineer
- R&D Manager
- IP Professional
- Industry Leading Data Capabilities
- Powerful AI technology
- Patent DNA Extraction
Browse by: Latest US Patents, China's latest patents, Technical Efficacy Thesaurus, Application Domain, Technology Topic, Popular Technical Reports.
© 2024 PatSnap. All rights reserved.Legal|Privacy policy|Modern Slavery Act Transparency Statement|Sitemap|About US| Contact US: help@patsnap.com