Super-resolution reconstruction method based on multi-path deep convolutional neural network
A super-resolution reconstruction and convolutional neural network technology, applied in the field of image processing, can solve problems such as inability to generate high-frequency details, inability to process complex image structures of natural images, etc.
- Summary
- Abstract
- Description
- Claims
- Application Information
AI Technical Summary
Problems solved by technology
Method used
Image
Examples
Embodiment Construction
[0069] Specific embodiments of the present invention are described in detail below, but it should be understood that the protection scope of the present invention is not limited by the specific embodiments.
[0070] Unless expressly stated otherwise, throughout the specification and claims, the term "comprise" or variations thereof such as "includes" or "includes" and the like will be understood to include the stated elements or constituents, and not Other elements or other components are not excluded.
[0071] (1) Prepare the training set. The general super-resolution network training set 91images and the 200images in the Berkeley segmentation data set were combined as the training set. The original training set was 291 natural pictures. In order to make full use of the training pictures, the training set was enhanced in the experiment. For each The training pictures are reduced by 0.9, 0.8, 0.7, and 0.6 times, and rotated by 90°, 180°, 270° and mirrored. Therefore, a natura...
PUM
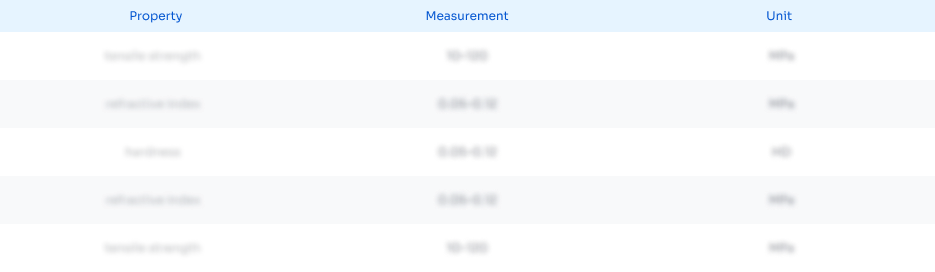
Abstract
Description
Claims
Application Information
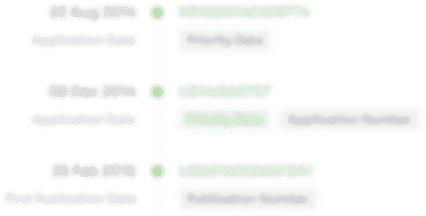
- R&D Engineer
- R&D Manager
- IP Professional
- Industry Leading Data Capabilities
- Powerful AI technology
- Patent DNA Extraction
Browse by: Latest US Patents, China's latest patents, Technical Efficacy Thesaurus, Application Domain, Technology Topic, Popular Technical Reports.
© 2024 PatSnap. All rights reserved.Legal|Privacy policy|Modern Slavery Act Transparency Statement|Sitemap|About US| Contact US: help@patsnap.com