Knowledge graph entity semantic space embedding method based on graph second-order similarity
A knowledge graph and similarity technology, which is applied in the field of knowledge graph entity semantic space embedding based on the second-order similarity of graphs, can solve the problems of unsatisfactory link prediction and classification feature experiments, and achieve a good embedding effect.
- Summary
- Abstract
- Description
- Claims
- Application Information
AI Technical Summary
Problems solved by technology
Method used
Image
Examples
Embodiment Construction
[0031] The present invention will be further described in detail with the help of drawings and examples below, but the embodiments of the present invention are not limited thereto.
[0032] The present invention is a representation learning method that comprehensively considers the first-order and second-order similarity of the graph structure in the knowledge graph, maps the entities and relationships in the knowledge graph into low-dimensional vectors, and uses the low-dimensional vectors to complete knowledge graphs and triplets Classification. Firstly, according to the first-order similarity of the graph structure encoded by the graph neural network, the neighbor entity vector is projected into the relationship matrix space directly connected to the entity, and then the average value of all neighbors projected into the relationship matrix space is calculated to represent the initial first-order similarity of the entity. Similarity vector, the initial first-order similarity...
PUM
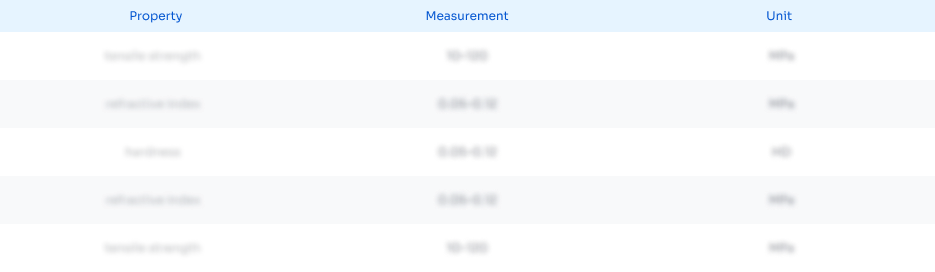
Abstract
Description
Claims
Application Information
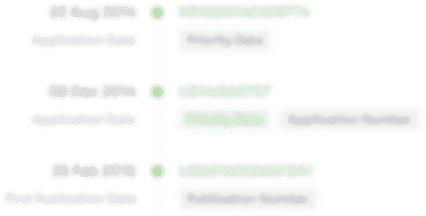
- Generate Ideas
- Intellectual Property
- Life Sciences
- Materials
- Tech Scout
- Unparalleled Data Quality
- Higher Quality Content
- 60% Fewer Hallucinations
Browse by: Latest US Patents, China's latest patents, Technical Efficacy Thesaurus, Application Domain, Technology Topic, Popular Technical Reports.
© 2025 PatSnap. All rights reserved.Legal|Privacy policy|Modern Slavery Act Transparency Statement|Sitemap|About US| Contact US: help@patsnap.com