Dexterous hand grabbing planning method based on four-stage convolutional neural networks
A convolutional neural network and dexterous hand technology, applied in the field of computer vision, can solve problems such as limited application, only considering gripper grasping planning, and inability to use grasping planning, achieving strong generalization ability and simple grasping planning And easy to operate, improve the effect of grasping ability
- Summary
- Abstract
- Description
- Claims
- Application Information
AI Technical Summary
Problems solved by technology
Method used
Image
Examples
Embodiment Construction
[0043] In this embodiment, the four-level convolutional neural network-based dexterous hand grasping planning method applied to the object grasping operation composed of a robot, a camera, and a target includes: acquiring grasping frame data sets and grasping gesture data Set, design a four-level convolutional neural network structure, obtain the depth map of the grasped part of the target, and determine the position and posture of the dexterous hand. Among them, for the four-level convolutional neural network, the first, second and third levels are used to detect the best grasping frame of the object, and obtain the depth map of the grasped part of the object; the fourth level network is based on the depth map of the grasped part and the dexterous hand The pose information of the dexterous hand is used to predict the grasping gesture of the dexterous hand. Specifically, proceed as follows:
[0044] Step 1: Obtain the grab frame dataset and grab gesture dataset:
[0045] Step ...
PUM
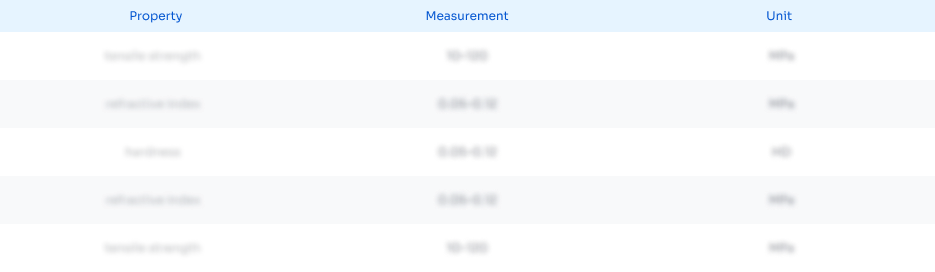
Abstract
Description
Claims
Application Information
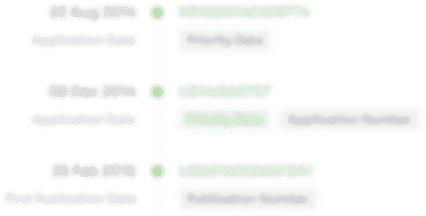
- R&D
- Intellectual Property
- Life Sciences
- Materials
- Tech Scout
- Unparalleled Data Quality
- Higher Quality Content
- 60% Fewer Hallucinations
Browse by: Latest US Patents, China's latest patents, Technical Efficacy Thesaurus, Application Domain, Technology Topic, Popular Technical Reports.
© 2025 PatSnap. All rights reserved.Legal|Privacy policy|Modern Slavery Act Transparency Statement|Sitemap|About US| Contact US: help@patsnap.com