Adaptive learning rate schedule in distributed stochastic gradient descent
A machine learning and gradient technology, applied in machine learning, computer components, character and pattern recognition, etc.
- Summary
- Abstract
- Description
- Claims
- Application Information
AI Technical Summary
Problems solved by technology
Method used
Image
Examples
Embodiment Construction
[0011] In describing the exemplary embodiments of the invention illustrated in the drawings, specific terminology will be employed for the sake of clarity. However, the invention is not intended to be limited to this description or to any particular term, and it is to be understood that each element includes all equivalents.
[0012] Exemplary embodiments of the present invention may utilize a distributed approach to perform stochastic gradient descent (SGD), where a central parameter server (PS) is used to manage SGD as multiple learner machine process gradients in parallel. The parameter server updates the model parameters based on the results of the processed gradients, and the learner machine can then use the updated model parameters in processing subsequent gradients. In this sense, stochastic gradient descent is performed in a distributed fashion, and thus the process can be called distributed stochastic gradient descent.
[0013] When performing distributed SGD, learne...
PUM
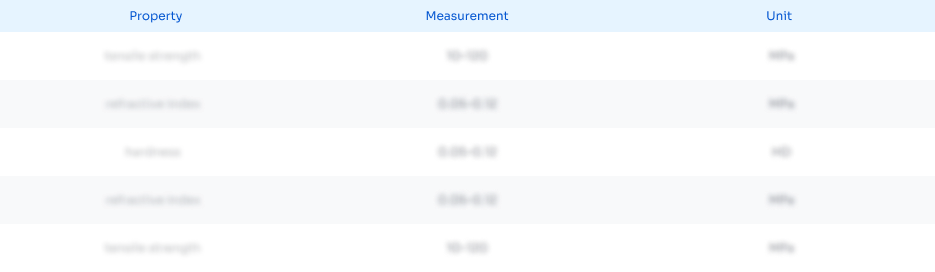
Abstract
Description
Claims
Application Information
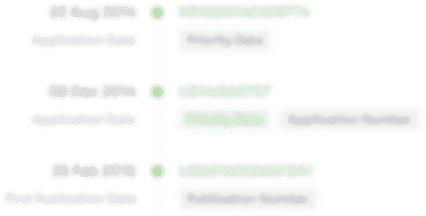
- R&D Engineer
- R&D Manager
- IP Professional
- Industry Leading Data Capabilities
- Powerful AI technology
- Patent DNA Extraction
Browse by: Latest US Patents, China's latest patents, Technical Efficacy Thesaurus, Application Domain, Technology Topic.
© 2024 PatSnap. All rights reserved.Legal|Privacy policy|Modern Slavery Act Transparency Statement|Sitemap