Human action recognition method based on sparse tensor local fisher discriminant analysis algorithm
A discriminant analysis and behavior technology, applied in character and pattern recognition, computing, computer components and other directions, can solve the problem of destroying the spatial correlation of images, and achieve the effect of improving the recognition rate of human behavior, ensuring sparsity, and good classification.
- Summary
- Abstract
- Description
- Claims
- Application Information
AI Technical Summary
Problems solved by technology
Method used
Image
Examples
Embodiment Construction
[0094] The technical solutions of the present invention will be further described below with reference to the accompanying drawings and embodiments.
[0095] A method for identifying human behavior based on a sparse tensor local Fisher discriminant analysis algorithm described in the present invention includes the following steps:
[0096] S1: Obtain the silhouette image sequence according to the Weizmann human behavior database, and construct tensor samples. 10 different actions in the human behavior database correspond to 10 types of tensor samples, and construct a training sample set and a test sample set according to the tensor samples ;
[0097] S2: The sparse tensor local Fisher discriminant analysis algorithm (STLFDA) is used to train according to the training sample set to obtain a sparse projection matrix group; the sparse projection matrix group can project the original tensor samples from a high-dimensional space to a low-dimensional tensor quantum space, In order ...
PUM
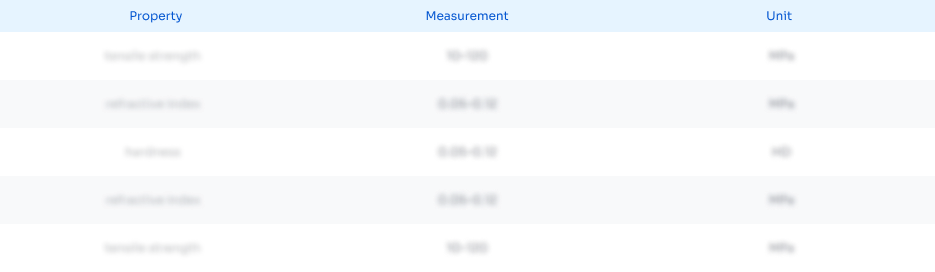
Abstract
Description
Claims
Application Information
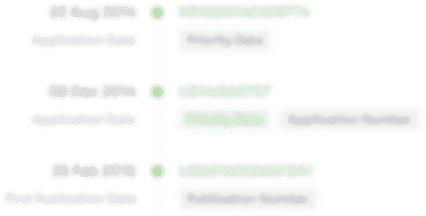
- R&D
- Intellectual Property
- Life Sciences
- Materials
- Tech Scout
- Unparalleled Data Quality
- Higher Quality Content
- 60% Fewer Hallucinations
Browse by: Latest US Patents, China's latest patents, Technical Efficacy Thesaurus, Application Domain, Technology Topic, Popular Technical Reports.
© 2025 PatSnap. All rights reserved.Legal|Privacy policy|Modern Slavery Act Transparency Statement|Sitemap|About US| Contact US: help@patsnap.com