Method for semi-supervised learning prediction of protein secondary structure
A semi-supervised learning, secondary structure technology, applied in neural learning methods, for analyzing two-dimensional or three-dimensional molecular structures, informatics, etc.
- Summary
- Abstract
- Description
- Claims
- Application Information
AI Technical Summary
Problems solved by technology
Method used
Image
Examples
Embodiment Construction
[0017] The present invention will be described in further detail below in conjunction with the accompanying drawings and specific embodiments. It should be understood that the specific embodiments described here are only used to explain the present invention, not to limit the present invention.
[0018] The invention provides a method for semi-supervised learning and prediction of protein secondary structure, such as figure 1 As shown, the details are as follows:
[0019] 1. Get the protein data set
[0020] First, a dataset needs to be obtained. In this example, the dataset used is the CullPDB dataset, which consists of 6133 proteins and each protein has 39900 features. 6133 proteins × 39900 features can be reshaped into 6133 proteins × 700 amino acids × 57 features. The following table is the protein secondary structure categories and the frequency of occurrence of each category:
[0021] Description of protein secondary structure classes and class frequencies in datase...
PUM
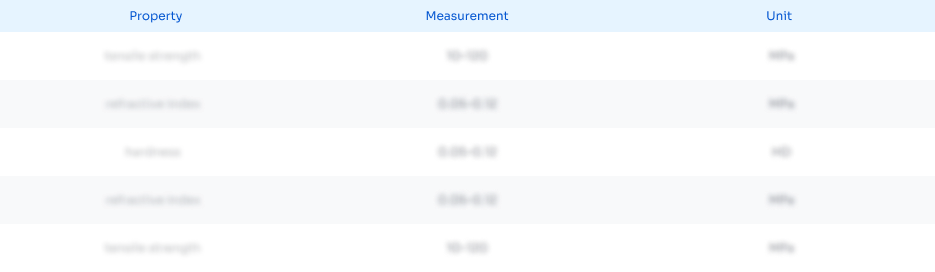
Abstract
Description
Claims
Application Information
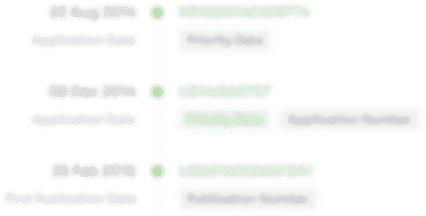
- R&D
- Intellectual Property
- Life Sciences
- Materials
- Tech Scout
- Unparalleled Data Quality
- Higher Quality Content
- 60% Fewer Hallucinations
Browse by: Latest US Patents, China's latest patents, Technical Efficacy Thesaurus, Application Domain, Technology Topic, Popular Technical Reports.
© 2025 PatSnap. All rights reserved.Legal|Privacy policy|Modern Slavery Act Transparency Statement|Sitemap|About US| Contact US: help@patsnap.com