A method for constructing a test set for text-level English-to-Chinese machine translation
A technology of machine translation and construction method, which is applied in the construction field of text-level English-to-Chinese machine translation test sets, and can solve the problems of no evaluation indicators
- Summary
- Abstract
- Description
- Claims
- Application Information
AI Technical Summary
Problems solved by technology
Method used
Image
Examples
example 1
[0088] Source language data:
[0089] Previous sentence: You rich guys think that money can buy anything.
[0090] Current Sentence: How right you are.
[0091] Target language data:
[0092] Previous sentence: You rich people always think that money can buy everything.
[0093] Current sentence: You are so right.
[0094] The text-level connective test set requires that the current sentence in the source language data contains one of five text-level connectives such as "as", "or", "while", "since", and "though", and the connection The part-of-speech of a word needs to satisfy one of "CC", "IN", and "WRB". Due to the diverse expressions of Chinese discourse-level connectives, we first automatically filter the sentence pairs that contain the corresponding meaning, and then take manual checks to satisfy the source language. Sentence pairs for each condition in the data but the target language data does not contain the corresponding connective, then check whether the informat...
example 2
[0097] Source language data:
[0098] Previous sentence: Everything is so difficult in life, for me.
[0099] Current Sentence: While for others it’s all child’s play.
[0100] Target language data:
[0101] Previous sentence: For me, everything in life is very difficult.
[0102] Current sentence: To others, it is like child's play.
[0103] Omit the test set to filter out the sentence pairs containing auxiliary verbs in the current sentence of the source language data by character matching, that is, including "do", "does", "can", "could", "should", "is", "am" ", "are", "may", and then the previous sentence of the source language data needs to contain verbs, that is, the parts of speech are "VC", "VE", "VV", and then check the current sentence in the target language data. The verbs in the previous sentence are consistent with the verbs in the previous sentence, and finally a certain number of test cases are selected to form the omitted test set.
[0104] Then check the v...
example 3
[0106] Source language data:
[0107] The previous sentence: You see, she doesn't know.
[0108] Current Sentence: Neither do I.
[0109] Target language data:
[0110] Previous sentence: Look, she doesn't know.
[0111] Current sentence: I don't know either.
[0112] Step 4: Manually check the selected test cases to correct translation errors.
[0113] Table 1: BLEU automatic scoring results
[0114] pronoun discourse-level connectives omit thumb 12.4 9.8 18.2 CADec 19.1 15.3 25.5 bert-nmt 13.9 12.7 19.1
[0115] It can be seen from Table 1: From the perspective of BLEU (Bilingual Evaluation Substitute) value, the CADec (Combined Context Decoder) model has the highest BLEU value on the three language phenomena, indicating that the model has the highest BLEU value on the three text-level language phenomena. The translation effect is the best, the BLEU value of the bert-nmt (neural machine translation with BERT fusion) model ran...
PUM
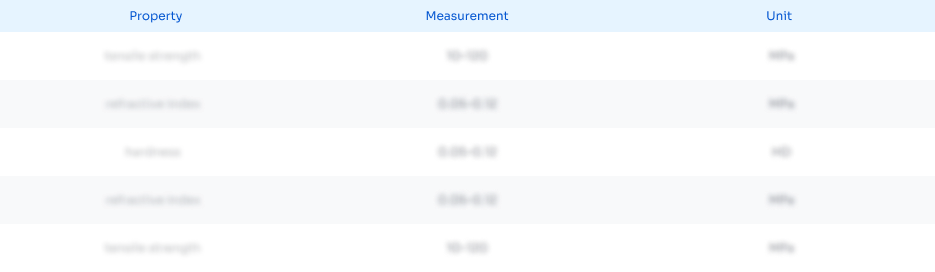
Abstract
Description
Claims
Application Information
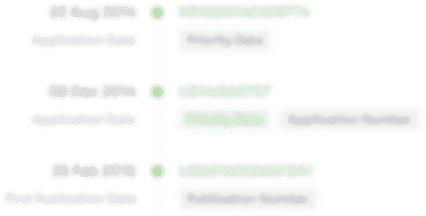
- R&D Engineer
- R&D Manager
- IP Professional
- Industry Leading Data Capabilities
- Powerful AI technology
- Patent DNA Extraction
Browse by: Latest US Patents, China's latest patents, Technical Efficacy Thesaurus, Application Domain, Technology Topic, Popular Technical Reports.
© 2024 PatSnap. All rights reserved.Legal|Privacy policy|Modern Slavery Act Transparency Statement|Sitemap|About US| Contact US: help@patsnap.com