Multilingual model training method based on cross-language self-training
A model training and multilingual technology, applied in speech analysis, speech recognition, instruments, etc., can solve the problem that the teacher's model cannot be decoded, and achieve the effect of enhancing the ability of semantic representation
- Summary
- Abstract
- Description
- Claims
- Application Information
AI Technical Summary
Problems solved by technology
Method used
Image
Examples
Embodiment Construction
[0026] The current unsupervised pre-training method has the limitation of insufficient semantic representation, while the supervised pre-training method has the limitation of insufficient data. Therefore, the present invention proposes a multilingual training method based on cross-language self-training, with the purpose of using a small amount of labeled data to enhance the semantic representation ability of the model on multilingual unlabeled data. The model pre-trained by this method can be used as a multilingual general initialization model and migrated to any low-resource language to improve the accuracy of the low-resource language ASR model.
[0027] The present invention proposes a model training method based on Cross-lingual Self-training (XLST). In the framework of this training model, we assume that frame-level speech representations have shared properties across languages. The method first trains an acoustic phoneme classifier on labeled data in a high-resource la...
PUM
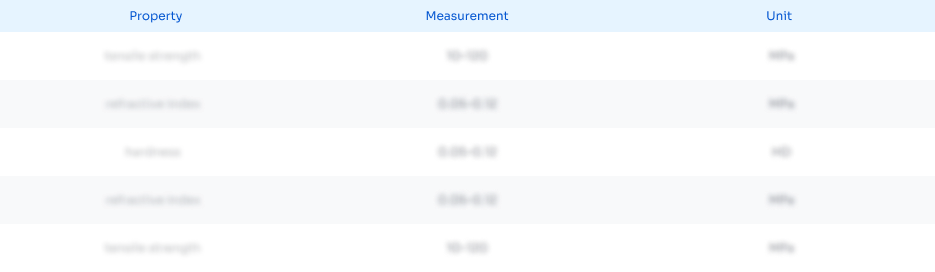
Abstract
Description
Claims
Application Information
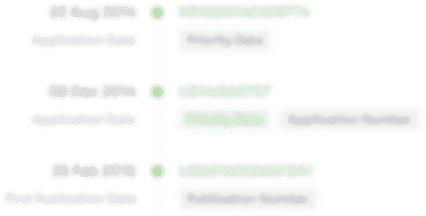
- R&D Engineer
- R&D Manager
- IP Professional
- Industry Leading Data Capabilities
- Powerful AI technology
- Patent DNA Extraction
Browse by: Latest US Patents, China's latest patents, Technical Efficacy Thesaurus, Application Domain, Technology Topic, Popular Technical Reports.
© 2024 PatSnap. All rights reserved.Legal|Privacy policy|Modern Slavery Act Transparency Statement|Sitemap|About US| Contact US: help@patsnap.com