Material performance prediction method based on dynamic selection training set
A technology for material properties and prediction methods, applied in computer material science, machine learning, instruments, etc., can solve the problems of time-consuming, labor-intensive, prediction accuracy errors, and large prediction errors of test data.
- Summary
- Abstract
- Description
- Claims
- Application Information
AI Technical Summary
Problems solved by technology
Method used
Image
Examples
Embodiment 1
[0030] The current machine learning method for predicting material performance cannot select a suitable training set, ignores the characteristic differences between the original data, and has a large prediction error for the test data at the edge of the cluster, and thus cannot accurately predict the test data. The present invention uses A method of dynamically selecting model training sets to predict the performance of material test data, that is, for each set of new material test data to be predicted, we select different numbers of specific similar data from the original database in real time as the training set. Train models to improve the accuracy of material performance predictions.
[0031] See figure 1 , the present embodiment provides a material performance prediction method based on dynamically selecting a training set, which mainly includes the following steps:
[0032] S101. Obtain the original material parameter data of each sample in the training database, use th...
PUM
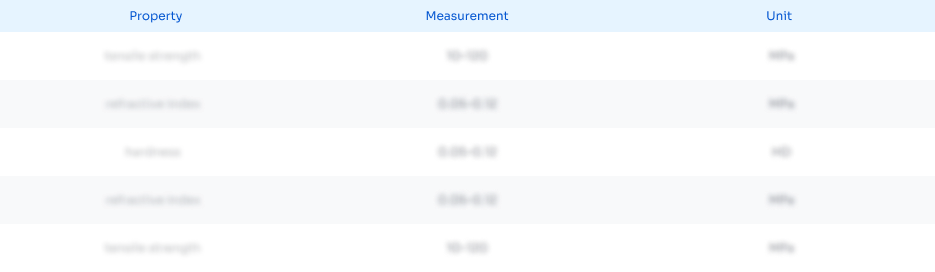
Abstract
Description
Claims
Application Information
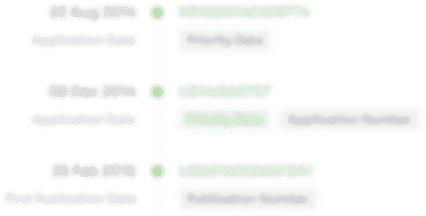
- R&D Engineer
- R&D Manager
- IP Professional
- Industry Leading Data Capabilities
- Powerful AI technology
- Patent DNA Extraction
Browse by: Latest US Patents, China's latest patents, Technical Efficacy Thesaurus, Application Domain, Technology Topic, Popular Technical Reports.
© 2024 PatSnap. All rights reserved.Legal|Privacy policy|Modern Slavery Act Transparency Statement|Sitemap|About US| Contact US: help@patsnap.com