Multiple output relaxation machine learning model
A machine learning model and multi-output technology, applied in machine learning, computing models, neural learning methods, etc., can solve problems such as inability to deal with the interdependence of different output components
- Summary
- Abstract
- Description
- Claims
- Application Information
AI Technical Summary
Problems solved by technology
Method used
Image
Examples
Embodiment Construction
[0028]Some embodiments described herein include methods employing multiple output relaxation (MOR) machine learning models to predict multiple interdependent output components of multiple output dependent (MOD) output decisions. The example methods disclosed herein can be used to solve the MOD problem.
[0029] As used herein, the term "multiple output dependency" or "MOD" refers to an output decision, or a problem with output decisions, that includes multiple output components that are interdependent in that each component depends not only on depends on the input and depends on other components. Some example MOD questions include, but are not limited to: 1) a mix of stocks to buy to balance a mutual fund given current stock market conditions; 2) a mix of players to add to a sports team's roster given an opposing team's current roster; And 3) The combination of shirt, pants, belt and shoes to wear given the current weather conditions. In each of these examples, each componen...
PUM
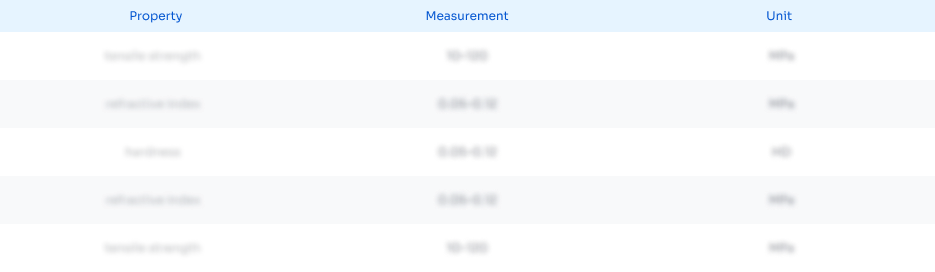
Abstract
Description
Claims
Application Information
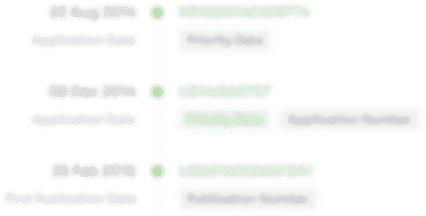
- R&D Engineer
- R&D Manager
- IP Professional
- Industry Leading Data Capabilities
- Powerful AI technology
- Patent DNA Extraction
Browse by: Latest US Patents, China's latest patents, Technical Efficacy Thesaurus, Application Domain, Technology Topic, Popular Technical Reports.
© 2024 PatSnap. All rights reserved.Legal|Privacy policy|Modern Slavery Act Transparency Statement|Sitemap|About US| Contact US: help@patsnap.com