Protein structure class prediction method based on weighted composition position vectors and support vector machine
A protein structure and support vector machine technology, which is applied in the field of protein structure prediction based on weighted composition position vectors, can solve the problems of missing amino acid residue sequence information, limiting the performance of prediction methods, and inability to distinguish protein sequences, so as to achieve good popularization and application. Value, richness of representational information, simple prediction method effect
- Summary
- Abstract
- Description
- Claims
- Application Information
AI Technical Summary
Benefits of technology
Problems solved by technology
Method used
Image
Examples
Embodiment 1
[0037] 1. Select the standard data set of protein structure
[0038] In this embodiment, two standard data sets of protein structures, Z277 and Z498, are selected as examples for protein prediction.
[0039] Z277 and Z498 two protein structure standard dataset information:
[0040] The Z277 dataset contains a total of 277 protein sequences, including 70 all-a (all-a) proteins, 61 all-b (all-b) proteins, 81 a / b-type proteins and 65 a+b-type proteins .
[0041] The Z498 data set contains a total of 498 protein sequences, including 107 all-a (all-a) proteins, 126 all-b (all-b) proteins, 136 a / b-type proteins and 129 a+b-type proteins .
[0042] 2. Analysis of amino acid composition
[0043] When amino acid composition is used to characterize protein sequences, the sequence information between amino acid residues in the sequence will be lost, and amino acid composition characterization methods cannot distinguish protein sequences with the same amino acid composition but differ...
PUM
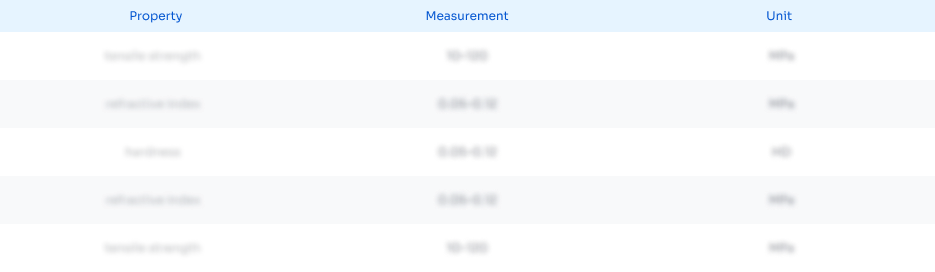
Abstract
Description
Claims
Application Information
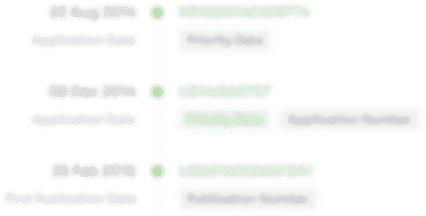
- R&D Engineer
- R&D Manager
- IP Professional
- Industry Leading Data Capabilities
- Powerful AI technology
- Patent DNA Extraction
Browse by: Latest US Patents, China's latest patents, Technical Efficacy Thesaurus, Application Domain, Technology Topic, Popular Technical Reports.
© 2024 PatSnap. All rights reserved.Legal|Privacy policy|Modern Slavery Act Transparency Statement|Sitemap|About US| Contact US: help@patsnap.com