Video super-resolution reconstruction method based on deep learning and self-similarity
A super-resolution reconstruction and self-similarity technology, which is applied in the field of video super-resolution reconstruction based on deep learning and self-similarity, can solve problems such as long training time, low reconstruction magnification, and poor reconstruction effect
- Summary
- Abstract
- Description
- Claims
- Application Information
AI Technical Summary
Problems solved by technology
Method used
Image
Examples
Embodiment Construction
[0049] In order to make the object, technical solution and advantages of the present invention clearer, the present invention will be described in further detail below in conjunction with specific embodiments and with reference to the accompanying drawings.
[0050]In order to make the method of the embodiment of the present invention easy to understand, and also to make the following discussion more convenient, some basic concepts are defined here first, and these basic concepts will not be described in detail below.
[0051] Temporal Neighborhood: If the sound is not considered, a video can be regarded as a sequence of video frames, and each video frame corresponds to a moment. The so-called temporal neighborhood refers to a neighborhood selected in the time dimension with the moment of a certain video frame as the center.
[0052] Spatial neighborhood: A video frame is a two-dimensional image. If you choose a point on the image, you can select a neighborhood in the video fr...
PUM
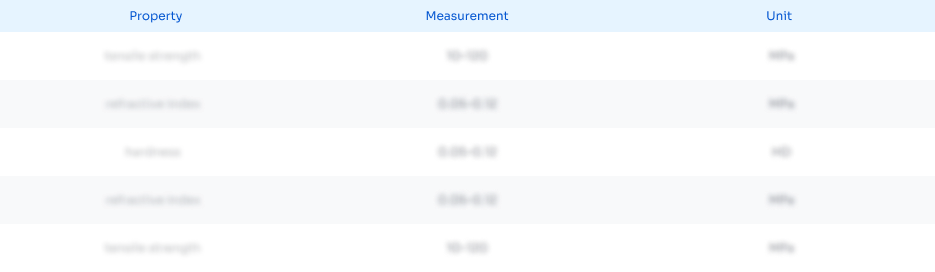
Abstract
Description
Claims
Application Information
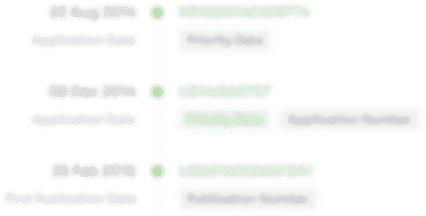
- R&D
- Intellectual Property
- Life Sciences
- Materials
- Tech Scout
- Unparalleled Data Quality
- Higher Quality Content
- 60% Fewer Hallucinations
Browse by: Latest US Patents, China's latest patents, Technical Efficacy Thesaurus, Application Domain, Technology Topic, Popular Technical Reports.
© 2025 PatSnap. All rights reserved.Legal|Privacy policy|Modern Slavery Act Transparency Statement|Sitemap|About US| Contact US: help@patsnap.com