Image super-resolution reconstruction method and electronic equipment based on gradual fusion of features
A technology of super-resolution reconstruction and feature fusion, which is applied in the image super-resolution reconstruction method of gradually fused features and in the field of electronic equipment, can solve the problems of low efficiency of model effective information extraction, limit the quality of neural network reconstructed images, etc., and achieve improvement Super-resolution reconstruction effect, reduction of useless information redundancy, effect of small computer memory
- Summary
- Abstract
- Description
- Claims
- Application Information
AI Technical Summary
Problems solved by technology
Method used
Image
Examples
Embodiment 1
[0053] according to figure 1 The super-resolution reconstruction network structure shown is used to build the model, the code uses the 3.7 version of python, and uses the pytorch framework. In terms of hardware, the CPU used for model training and testing is Inteli9, with 128G of memory, and the graphics card is NVIDIA 2080ti with 11G of memory.
[0054] In this embodiment, the first feature extraction module 3 is implemented using a convolution layer with a convolution kernel size of 3*3, and the structure of the second feature extraction module 4 is as follows figure 2 As shown, the local dimensionality reduction layer 44 is a convolution layer with a convolution kernel size of 1*1. The second feature extraction module 4 and the third feature extraction module 5 have a one-to-one correspondence relationship, and the number of the second feature extraction module 4 and the third feature extraction module 5 are both three. After the feature map input into the second feature...
Embodiment 2
[0065] As a comparison, in this embodiment, on the basis of Embodiment 1, the channel attention module 45 and the AM pooling layer 452 are separately removed, and the remaining parts and experimental conditions are exactly the same as those in Embodiment 1. The comparison of experimental results is shown in the following table:
[0066] Model gain set5 set14 BSDS100 Example 1 2 39.13 / 0.9644 32.76 / 0.9074 33.57 / 0.9395 Model A 2 38.26 / 0.9602 32.41 / 0.9031 33.35 / 0.9390 Model B 2 38.57 / 0.9609 32.62 / 0.9371 33.35 / 0.9395 Example 1 4 33.16 / 0.9013 28.05 / 0.7448 27.53 / 0.8195 Model A 4 32.73 / 0.9004 27.90 / 0.7441 27.22 / 0.8171 Model B 4 32.92 / 0.9010 27.90 / 0.7440 28.25 / 0.8188
[0067]In the above table, Model A is obtained after removing the channel attention module 45 alone on the basis of Example 1, and Model B is obtained after removing the AM pooling layer 452 on the basis of Example 1 and leaving the variance pooling...
Embodiment 3
[0069] On the basis of the image super-resolution reconstruction network in embodiment 1, a jump connection module 9 is added for comparative experiments, and its structure is as follows Image 6 Shown, all the other parts are exactly the same as in Example 1.
[0070] In this embodiment, the original feature map is generated after the first skip-connected convolutional layer 91 and the fifth ReLU activation function 96 to generate the first skip-connected feature map, and the last feature map output by the second feature extraction module 4 is extracted and passed through the second After the skip-connected convolution layer 92 and the sixth ReLU activation function 97, a second skip-connected feature map is generated, and after the first skip-connected feature map and the second skip-connected feature map are spliced, they pass through the third skip-connected convolution layer 94 in sequence , the second sub-pixel convolution layer 93, the fourth skip convolution layer 95 a...
PUM
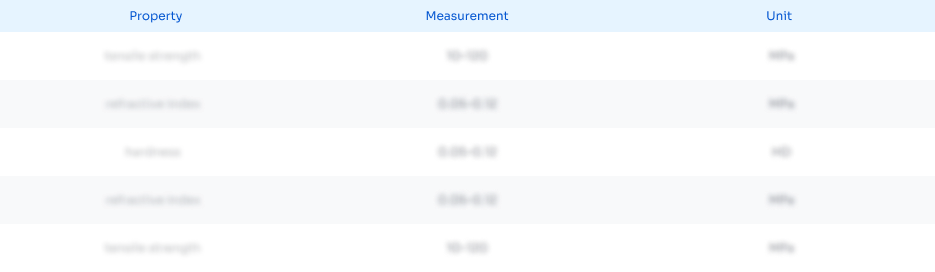
Abstract
Description
Claims
Application Information
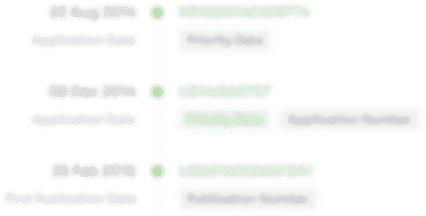
- R&D
- Intellectual Property
- Life Sciences
- Materials
- Tech Scout
- Unparalleled Data Quality
- Higher Quality Content
- 60% Fewer Hallucinations
Browse by: Latest US Patents, China's latest patents, Technical Efficacy Thesaurus, Application Domain, Technology Topic, Popular Technical Reports.
© 2025 PatSnap. All rights reserved.Legal|Privacy policy|Modern Slavery Act Transparency Statement|Sitemap|About US| Contact US: help@patsnap.com