Population counting method based on sub-module technology and semi-supervised learning
A semi-supervised learning and crowd counting technology, applied in the field of image and video-based crowd counting, which can solve problems such as inability to select samples, redundant information, and failure to consider the amount of information.
- Summary
- Abstract
- Description
- Claims
- Application Information
AI Technical Summary
Problems solved by technology
Method used
Image
Examples
experiment example 1
[0072] Experimental Example 1: Regression Algorithm Prediction Performance
[0073] This part of the experiment shows the error of different regression methods when randomly choosing labeled samples. As comparison methods, we choose Gaussian process regression with supervised learning, semi-supervised elastic net based and semi-supervised ridge regression. Table 1 shows the comparison of the regression algorithm of the present invention and the three comparison methods on the three datasets. It can be seen that on the UCSD and Fudan datasets, the performance of the present invention is the best, and on the Mall dataset, the performance of the present invention is very close to that of the semi-supervised elastic net.
experiment example 2
[0074] Experimental example 2: The impact of choosing different similarity measures on the model
[0075] Table 2 shows the performance changes of the regression model on the three data sets when using different similarity measures (ie, the weight ∈ of temporal similarity takes different values). It can be seen that on all datasets, using unlabeled samples can improve the prediction performance of the model; when using different similarity measures, it will have different effects on performance. If both temporal and spatial similarity are used (weight ∈ via Cross-validation obtained), the model can obtain the lowest prediction error.
experiment example 3
[0076] Experimental Example 3: Subplot Sample Selection Algorithm
[0077] Table 3 shows the effect of the subtype sample selection algorithm of the present invention. For comparison, k-means clustering and m-center point methods were selected. The k-means clustering method firstly clusters all samples, and then randomly selects samples from each cluster; the m-center point method first calculates the Laplacian matrix of the samples, and then obtains multiple clusters through spectral clustering, Finally select center point samples from each cluster.
[0078] Compared with Table 1, it can be found that for the UCSD data set, the samples selected by the semi-supervised elastic net and the sub-model technology of the present invention greatly improve the model performance; for the rest of the data sets, only the sub-model technology of the present invention can significantly improve the performance. In particular, the Mall dataset illustrates the applicability of the present i...
PUM
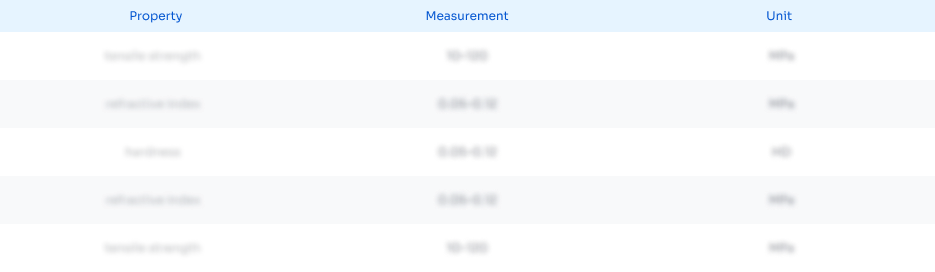
Abstract
Description
Claims
Application Information
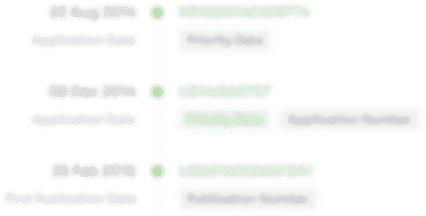
- R&D
- Intellectual Property
- Life Sciences
- Materials
- Tech Scout
- Unparalleled Data Quality
- Higher Quality Content
- 60% Fewer Hallucinations
Browse by: Latest US Patents, China's latest patents, Technical Efficacy Thesaurus, Application Domain, Technology Topic, Popular Technical Reports.
© 2025 PatSnap. All rights reserved.Legal|Privacy policy|Modern Slavery Act Transparency Statement|Sitemap|About US| Contact US: help@patsnap.com