Maxout multi-convolutional neural network fusion face recognition method and system
A convolutional neural network and face recognition system technology, applied in the field of image processing, can solve problems such as uneven classification of positive and negative samples, increased network training time, and different performance, so as to reduce the uneven performance of positive and negative samples and improve human performance. Face recognition accuracy, the effect of improving training speed
- Summary
- Abstract
- Description
- Claims
- Application Information
AI Technical Summary
Problems solved by technology
Method used
Image
Examples
Embodiment 1
[0030] The present invention is a kind of face recognition method based on Maxout multi-convolutional neural network fusion, see figure 1 , including the following steps:
[0031] Assume that the application environment of the face recognition method based on Maxout multi-convolutional neural network fusion proposed by the present invention is the entrance of a railway station, and the purpose is to identify the face image detected by the surveillance camera and the face image of the criminal suspect provided by the public security department , to achieve this, the implementation steps include:
[0032] Prepare training data:
[0033] (1a) Collect the face images detected by the surveillance camera at the entrance of the railway station, and use the collected face images as the training database D 0 .
[0034] (1b) From the training database D 0 Select an image, use the regression tree combination algorithm and affine transformation to perform face alignment correction on ...
Embodiment 2
[0042] The face recognition method based on Maxout multi-convolutional neural network fusion is the same as embodiment 1
[0043] Wherein the process of constructing fusion network based on Maxout in step (2) includes:
[0044] (2a) Build the first convolutional neural network, see figure 2 , figure 2 (a) is the first convolutional neural network structure diagram, which includes an input layer, 5 convolutional pooling layers, 2 fully connected layers, and an output layer in sequence according to the direction of data flow. figure 2 (b) is a schematic diagram of the convolutional pooling layer structure, where the convolutional pooling layer includes 2 convolutional layers with a convolution kernel size of 3×3, 2 Relu activation layers, and a Max pooling layer. In this example, two convolutional layers are connected alternately with two activation layers. The first is the convolutional layer; the next output is the Max pooling layer. The Max pooling layer can be replaced...
Embodiment 3
[0049] The face recognition method based on Maxout multi-convolutional neural network fusion is the same as embodiment 1-2, wherein the method utilizing Maxout in step (2c) fuses the convolutional neural network in step (2a) and step (2b) , obtain the fused convolutional neural network, comprising the following steps:
[0050] (2c1) Intercept the input layer, 5 convolutional pooling layers and the first fully connected layer in the first convolutional neural network constructed in step (2a) to obtain the subnetwork S 1 .
[0051] In this example from figure 2 In the first convolutional neural network structure shown in (a), all layer structures from the input layer to the first fully connected layer are sequentially selected according to the data flow direction to form a subnetwork S 1 .
[0052] (2c2) Intercept the input layer, 5 convolutional pooling layers and the first fully connected layer in the second convolutional neural network constructed in step (2b) to obtain t...
PUM
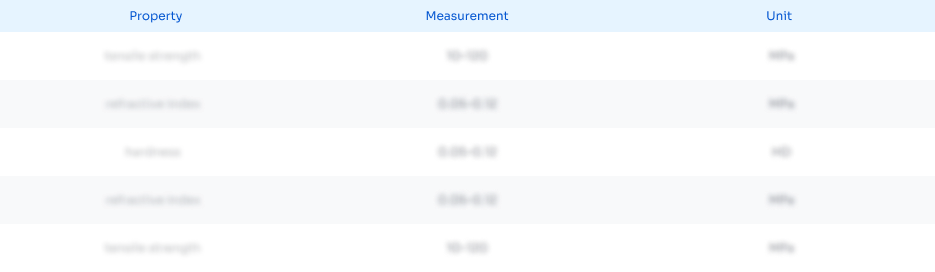
Abstract
Description
Claims
Application Information
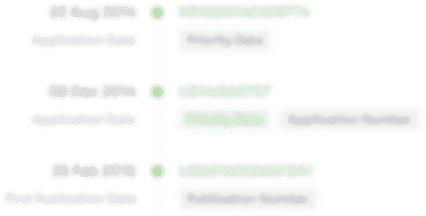
- R&D
- Intellectual Property
- Life Sciences
- Materials
- Tech Scout
- Unparalleled Data Quality
- Higher Quality Content
- 60% Fewer Hallucinations
Browse by: Latest US Patents, China's latest patents, Technical Efficacy Thesaurus, Application Domain, Technology Topic, Popular Technical Reports.
© 2025 PatSnap. All rights reserved.Legal|Privacy policy|Modern Slavery Act Transparency Statement|Sitemap|About US| Contact US: help@patsnap.com