Optimizing multi-class image classification using patch features
A block, image technology, applied in instrumentation, computing, character and pattern recognition, etc., can solve problems such as non-scalability
- Summary
- Abstract
- Description
- Claims
- Application Information
AI Technical Summary
Problems solved by technology
Method used
Image
Examples
Embodiment Construction
[0019] Computer vision object (e.g., people, animals, landmarks, etc.), texture, and / or scene classification (e.g., photos, videos, etc.) in images may be useful for including photo and / or video recognition, image search, product related search, etc. Current classification methods include training classifiers based on supervised or labeled data. This approach is not scalable or extensible. Furthermore, current classification methods exploit local image features (e.g., HOG) to learn common sense knowledge (e.g., eyes are part of a person) or specific sub-labels of a general label (e.g., a general label for horse includes subclasses of brown horse, riding horse, etc. Label). However, using local image features (eg, HOG) is computationally intensive. That said, current data mining techniques require a substantial investment in computer resources and are not scalable and / or scalable.
[0020] The technique described in this paper optimizes multi-class image classification by ex...
PUM
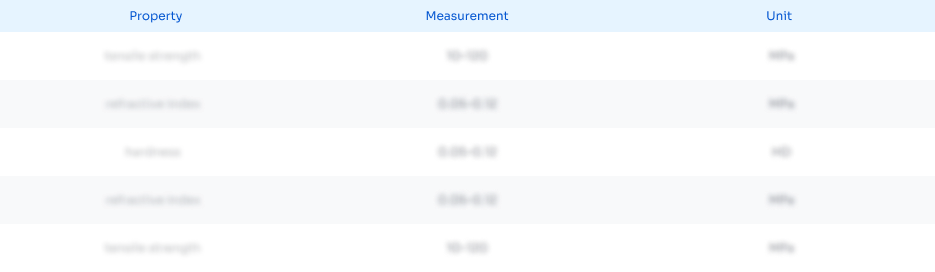
Abstract
Description
Claims
Application Information
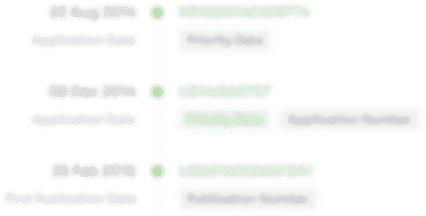
- Generate Ideas
- Intellectual Property
- Life Sciences
- Materials
- Tech Scout
- Unparalleled Data Quality
- Higher Quality Content
- 60% Fewer Hallucinations
Browse by: Latest US Patents, China's latest patents, Technical Efficacy Thesaurus, Application Domain, Technology Topic, Popular Technical Reports.
© 2025 PatSnap. All rights reserved.Legal|Privacy policy|Modern Slavery Act Transparency Statement|Sitemap|About US| Contact US: help@patsnap.com