Human action recognition method based on double layers of conditional random fields
A conditional random field and recognition method technology, applied in character and pattern recognition, computer components, instruments, etc., can solve the problems of high-order correlation, complex joint distribution modeling and recognition accuracy without considering the potential structure of human behavior state at the same time. low level problem
- Summary
- Abstract
- Description
- Claims
- Application Information
AI Technical Summary
Problems solved by technology
Method used
Image
Examples
Embodiment Construction
[0042] Below in conjunction with accompanying drawing, technical scheme of the present invention is described in further detail:
[0043] In order to solve the problems raised in the background technology, the present invention introduces a double-layer conditional random field model (DL-CRFs), which simultaneously captures the latent structure inside the human behavior state and the high-order Correlation.
[0044] Such as figure 1 Shown is the flow chart schematic diagram of the human behavior recognition method based on double-layer conditional random field of the present invention:
[0045] Step A, obtaining RGB-D training video samples containing human behavior RGB video information and depth information, and dividing each training video sample into a plurality of continuous video segments.
[0046] Step B, feature extraction: use OpenNI to extract the human body skeleton structure information of the subject of the action from the acquired depth information. Combining ...
PUM
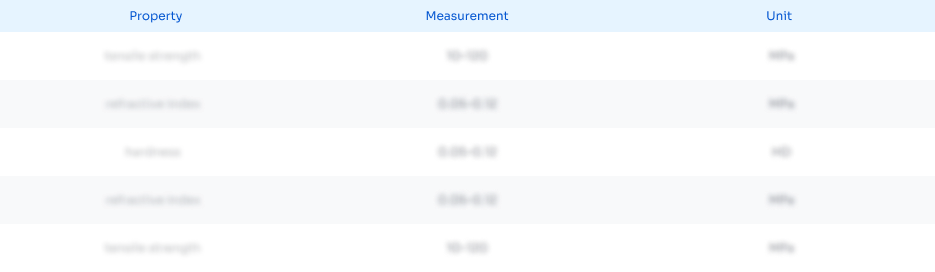
Abstract
Description
Claims
Application Information
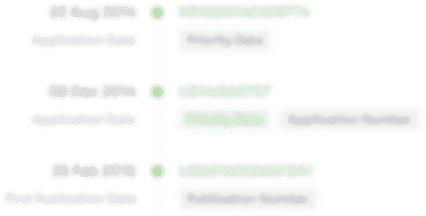
- R&D Engineer
- R&D Manager
- IP Professional
- Industry Leading Data Capabilities
- Powerful AI technology
- Patent DNA Extraction
Browse by: Latest US Patents, China's latest patents, Technical Efficacy Thesaurus, Application Domain, Technology Topic.
© 2024 PatSnap. All rights reserved.Legal|Privacy policy|Modern Slavery Act Transparency Statement|Sitemap