A Collaborative Filtering Method Fused with Content and Behavior for Personalized Recommendations
A collaborative filtering and behavioral technology, applied in data processing applications, character and pattern recognition, buying and selling/lease transactions, etc., can solve problems such as dependencies and data sparsity, improve efficiency and real-time performance, improve calculation accuracy, and improve recommendation. quality effect
- Summary
- Abstract
- Description
- Claims
- Application Information
AI Technical Summary
Problems solved by technology
Method used
Image
Examples
Embodiment
[0078] Such as figure 1 As shown, a collaborative filtering method for fusion content and behavior for personalized recommendation, the method includes the following steps:
[0079] (1) Feature input: feature input includes item-attribute matrix representing item content and user-item browsing matrix, user-item collection matrix, user-item purchase matrix, item evaluation label matrix and user-item rating matrix representing user behavior ;
[0080] (2) Content-based item clustering: combine item-attribute matrix and item evaluation label matrix, calculate item similarity, and cluster items;
[0081] (3) Rating prediction and feature filling: For the unrated items in the user-item rating matrix, find the nearest neighbor items in the clusters with high similarity to the unrated items, and according to the ratings of the nearest neighbor items, unrated The item performs rating prediction and populates the user-item rating matrix;
[0082] (4) Behavior-based user clustering: ...
PUM
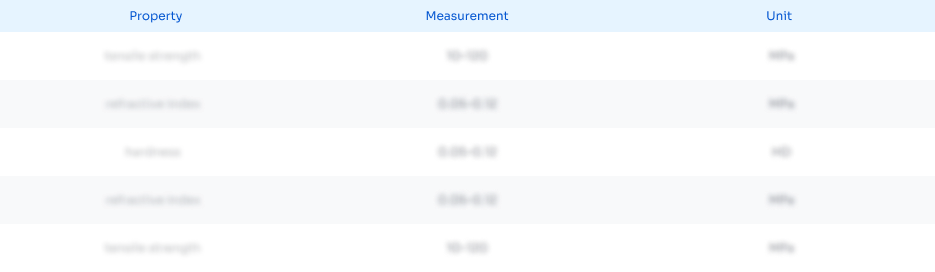
Abstract
Description
Claims
Application Information
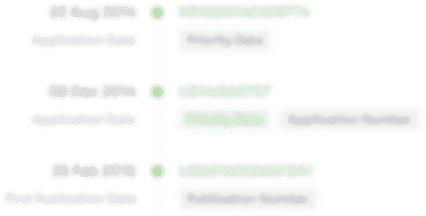
- R&D
- Intellectual Property
- Life Sciences
- Materials
- Tech Scout
- Unparalleled Data Quality
- Higher Quality Content
- 60% Fewer Hallucinations
Browse by: Latest US Patents, China's latest patents, Technical Efficacy Thesaurus, Application Domain, Technology Topic, Popular Technical Reports.
© 2025 PatSnap. All rights reserved.Legal|Privacy policy|Modern Slavery Act Transparency Statement|Sitemap|About US| Contact US: help@patsnap.com