A Clustering Method of User Preference and Distance Weighted Integrating Time Factor
A clustering method and distance weighting technology, applied in other database clustering/classification, data processing applications, special data processing applications, etc., can solve problems such as user cold start data sparsity, to solve data sparsity, solve interest Migrate, resolve cold start effects
- Summary
- Abstract
- Description
- Claims
- Application Information
AI Technical Summary
Problems solved by technology
Method used
Image
Examples
example 1
[0107] We verify the correctness and effectiveness of this algorithm through experiments, and verify the performance of the algorithm by comparing it with related algorithms. This experiment selected the 100K MovieLens dataset, which was collected by the GroupLens research team at the University of Minnesota. The file u.data included 100,000 ratings and timestamps for 1,682 movies by 943 users. Each user has at least 20 ratings, and the range of ratings is an integer from 1 to 5. The larger the value, the more the user likes the movie. This application mainly uses the mean absolute error (MAE) and F-Measure to analyze the experimental results.
[0108] The mean absolute error (MAE) is used to evaluate the degree of deviation between the user's predicted score and the actual score for an item. The smaller the value of MAE, the smaller the deviation and the better the recommendation effect. The formula is as follows:
[0109]
[0110] in: and Respectively represent the u...
PUM
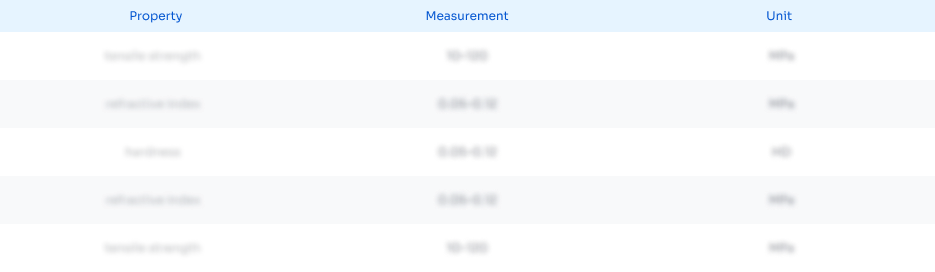
Abstract
Description
Claims
Application Information
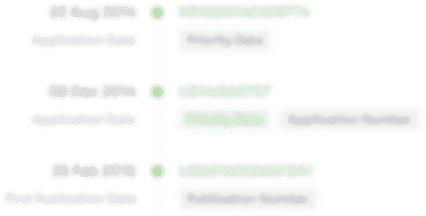
- Generate Ideas
- Intellectual Property
- Life Sciences
- Materials
- Tech Scout
- Unparalleled Data Quality
- Higher Quality Content
- 60% Fewer Hallucinations
Browse by: Latest US Patents, China's latest patents, Technical Efficacy Thesaurus, Application Domain, Technology Topic, Popular Technical Reports.
© 2025 PatSnap. All rights reserved.Legal|Privacy policy|Modern Slavery Act Transparency Statement|Sitemap|About US| Contact US: help@patsnap.com