Method for generating text based on pre-trained structured data
A structured data and pre-training technology, applied in neural learning methods, electrical digital data processing, special data processing applications, etc., can solve problems such as low accuracy of text generation and failure to consider the inherent implicit relationship of data
- Summary
- Abstract
- Description
- Claims
- Application Information
AI Technical Summary
Problems solved by technology
Method used
Image
Examples
specific Embodiment approach 1
[0053] Specific implementation mode one: combine figure 1 Describe this embodiment, the specific process of a method for generating text based on pre-trained structured data in this embodiment is:
[0054] The specific implementation is carried out on the NBA game data set rotowire. The NBA game Rotowire data set was proposed by the Harvard University Natural Language Processing Research Group in the "Challenges in Data-to-document Generation" paper work at the 2017 EMNLP conference. The data set consists of 4853 NBA games, and each game corresponds to a news report published by a reporter.
[0055] Construct the digital modeling pre-training target by artificially writing rules: due to the logical relationship of addition, subtraction, multiplication and division between data in the table information, that is, the total score of the team is composed of the scores of all the players of the team, or the total score of the players is composed of The team's four quarters are com...
specific Embodiment approach 2
[0115] Specific embodiment 2: The difference between this embodiment and specific embodiment 1 is that in the step 2, the model pre-training coding part is performed, and all the triple information obtained in step 1 is input into the pre-training model for entity relationship modeling to obtain The row vector row after performing mean pooling on all records in the same row in the table i (the same row in the table belongs to an entity, and the overall representation of the entity is obtained);
[0116] An example is as follows: Player A scored 16 points, 10 rebounds, and 4 assists in a game. The attributes of the entity of all A players are one row. Assume that the i-th row is the data of the A player, and the j-th The attribute is the score, ie r i,j Indicates that player A scored 16 points in this game, and the final modeling goal is to hope that the vector of scoring 16 integrates the information of all the data of player A, that is, to measure whether the score of 16 poi...
specific Embodiment approach 3
[0132] Specific implementation mode 3: The difference between this implementation mode and specific implementation mode 1 or 2 is that, in the step 3, to generate the @ hidden calculation sequence, the decoder needs to decode at each moment to generate the composition calculation sequence content; At time t of a decoding, there are two ways to obtain text through decoding, one is to copy from 602 triples, that is, the copy probability, and the other is to select a word from the vocabulary to generate, that is, the generation probability;
[0133] By generating text (vocabulary generation) or copying the value of the triplet (triple copy) to form the calculation sequence of @, until all of them are input into the pre-training model, and retaining the parameters of the pre-training model, retaining the parameters is equivalent to retaining the model through The ability obtained by pre-training; the process is:
[0134] Pass the hidden layer of the decoder LSTM at the current mom...
PUM
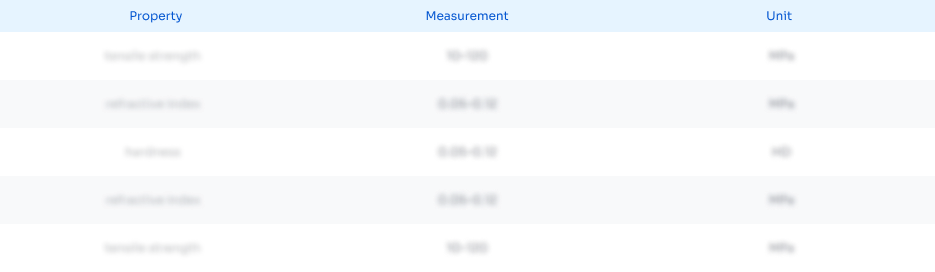
Abstract
Description
Claims
Application Information
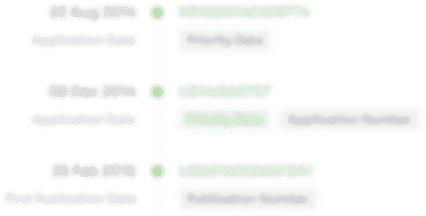
- R&D Engineer
- R&D Manager
- IP Professional
- Industry Leading Data Capabilities
- Powerful AI technology
- Patent DNA Extraction
Browse by: Latest US Patents, China's latest patents, Technical Efficacy Thesaurus, Application Domain, Technology Topic, Popular Technical Reports.
© 2024 PatSnap. All rights reserved.Legal|Privacy policy|Modern Slavery Act Transparency Statement|Sitemap|About US| Contact US: help@patsnap.com