Multi-instance natural scene text detection method based on relevance level residual errors
A text detection, natural scene technology, applied in the fields of deep learning, computer vision and text detection, can solve the problem of poor detection effect of small text area, loss function can not well evaluate the actual regression of text detection frame, etc., to reduce parameters The amount of calculation, the effect of improving text detection accuracy, and improving performance
- Summary
- Abstract
- Description
- Claims
- Application Information
AI Technical Summary
Problems solved by technology
Method used
Image
Examples
Embodiment Construction
[0052] Below in conjunction with accompanying drawing, the present invention is further described:
[0053] see figure 1 , the present invention comprises the following steps:
[0054] Step 101, using a camera to acquire image data or directly uploading image data as image input.
[0055] Step 102, use the feature extraction network based on the correlation residual to extract the features of the original input image, and obtain the combined sizes of the coarse-grained and fine-grained images as 1 / 32, 1 / 16, 1 / 8, 1 / 4 feature map f 1 , f 2 , f 3 , f 4 , these multi-scale feature maps respectively represent rich feature information from low-level to high-level.
[0056] Step 103, reverse step-by-step feature fusion from the feature map f 1 start, turn to f 1 , f 2 , f 3 , f 4 Upsampling and feature splicing are carried out, and finally a multi-scale fusion feature map with a size of 1 / 4 of the original input image is generated.
[0057] Step 104, perform text region...
PUM
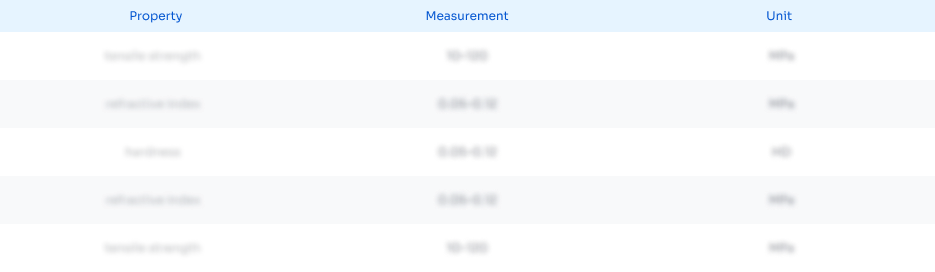
Abstract
Description
Claims
Application Information
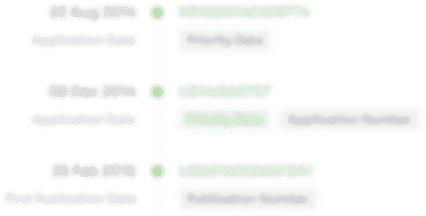
- R&D Engineer
- R&D Manager
- IP Professional
- Industry Leading Data Capabilities
- Powerful AI technology
- Patent DNA Extraction
Browse by: Latest US Patents, China's latest patents, Technical Efficacy Thesaurus, Application Domain, Technology Topic.
© 2024 PatSnap. All rights reserved.Legal|Privacy policy|Modern Slavery Act Transparency Statement|Sitemap